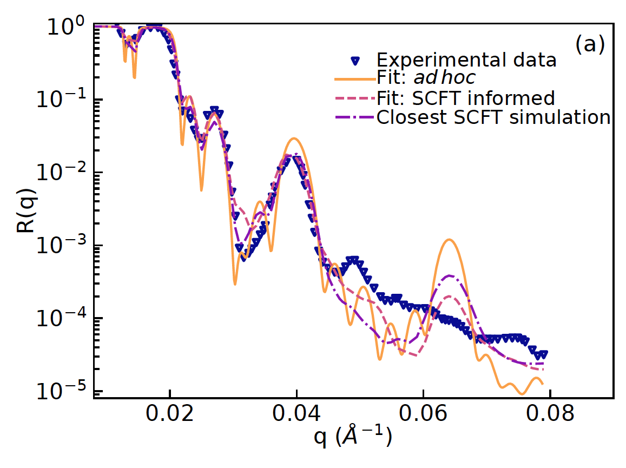
Neutron reflectivity vs. wavevector (q) for a film containing poly(dS-b-BMA). Two models containing multi-layers were constructed to fit experimental data: (1) ad hoc and (2) using information obtained from SCFT for number and thickness of microphase-separated domains. Reflectivity computed from SLD profile and constructed from SCFT simulation using three chi parameters closest to predicted parameters is also shown.
Scientific Achievement
A computational approach was developed that combines self consistent field theory (SCFT) and neural networks to extract physical parameters from specular neutron reflectivity data of thin block copolymers.
Significance and Impact
Results demonstrate the potential of combining modeling with machine learning to interpret specular reflectivity data of thin block copolymers. This development paves the way for solving the “inverse problem,” where experimental data is used to deduce an accurate physical model.
Research Details
- Thousands of simulated scattering length density curves were used to train neural networks (see Figure)
- Networks learn the relation between curves and physical parameters, and predict the physical parameters associated with an experimental curve not seen during training
- Interpretation of the results can be improved by increasing the model’s dimensionality
D. Eby, M. Jakowski, V. Lauter, M. Doucet, P. Ganesh, M. Fuentes-Cabrera, and R. Kumar, Nanoscale, accepted (2023). DOI:10.1039/D2NR07173H
Work conducted at the Center for Nanophase Materials Sciences and the Spallation Neutron Source.