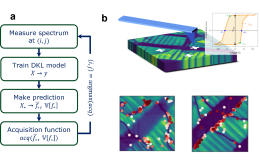
Scientific Achievement
A machine learning (ML) framework was developed that actively discovers relationships between local domain structure and material properties encoded in spectra.
Significance and Impact
Approach combines the power of ML methods to discover correlations in multidimensional data sets with human-level analysis and insights encoded in the acquisition function. Workflow is universal and can be extended to other spectroscopic and imaging methods in scanning probe and electron microscopy.
Research Details
Workflow learns structure-property relationships on-the-fly and uses this ‘knowledge’ to select the next measurement point.
Piezoresponse force microscopy is used for active discovery of relationships between local domain structures and polarization switching characteristics in ferroelectrics.
Y. Liu, K.P. Kelley, R.K. Vasudevan, H. Funakubo, M.A. Ziatdinov , and S.V. Kalinin, Nature Machine Intelligence (2022). DOI: 10.1038/s42256-022-00460-0 Work was performed at the Center for Nanophase Materials Sciences and the Tokyo Institute of Technology.