Invention Reference Number
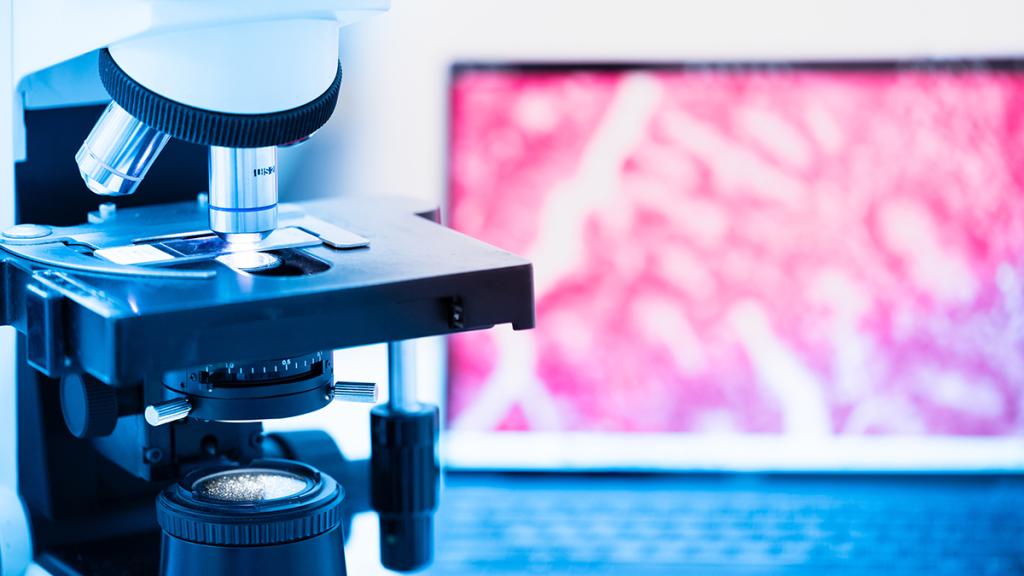
Description
The core of this technology lies in its ability to predict a spectral image from an optical image of a patient's tissue sample using a machine learning model. Different models are tailored for various cancers, trained with numerous image pairs from respective modalities, enhancing the system's predictive power. Subsequently, cancer labels are predicted from these spectral images through another layer of machine learning analysis, incorporating manual cancer labels for training. This dual-model approach effectively bridges the gap between optical imaging, commonly used in pathology, and spectral imaging, rich in molecular information, thereby providing a comprehensive view of the tissue's health status.
Benefits
- Increased accuracy in cancer detection and classification
- Early diagnosis through non-invasive methods
- Reduction in diagnostic costs by minimizing the need for multiple tests
- Customizable to various cancer types for broad application
Applications and Industries
- Diagnostic laboratories and pathology for enhanced cancer diagnosis
- Research institutions studying cancer's molecular basis
- Biotechnology and pharmaceutical companies developing targeted cancer therapies
Related ORNL Technologies
- Correlative Multimodal Chemical Imaging via Machine Learning
- Pan-Sharpening for Microscopy
- Science-Driven Automated Experiments
- Hypothesis Learning in Automated Experiments
- Human-in-the-Loop Machine Learning for Automated Experiments
Contact
To learn more about this technology, email partnerships@ornl.gov or call 865-574-1051.