Filter Results
Related Organization
- Biological and Environmental Systems Science Directorate (23)
- Computing and Computational Sciences Directorate (35)
- Energy Science and Technology Directorate (217)
- Fusion and Fission Energy and Science Directorate (21)
- Information Technology Services Directorate (2)
- Isotope Science and Enrichment Directorate (6)
- National Security Sciences Directorate (17)
- Neutron Sciences Directorate (11)
- Physical Sciences Directorate (128)
- User Facilities (27)
Researcher
- Adam M Guss
- Blane Fillingim
- Brian Post
- Josh Michener
- Lauren Heinrich
- Peeyush Nandwana
- Sudarsanam Babu
- Thomas Feldhausen
- Xiaohan Yang
- Yousub Lee
- Alexander I Wiechert
- Alex Walters
- Andrzej Nycz
- Austin Carroll
- Carrie Eckert
- Clay Leach
- Costas Tsouris
- Debangshu Mukherjee
- Gerald Tuskan
- Gs Jung
- Gyoung Gug Jang
- Ilenne Del Valle Kessra
- Isaiah Dishner
- Jay D Huenemann
- Jeff Foster
- Joanna Tannous
- John F Cahill
- Kyle Davis
- Liangyu Qian
- Md Inzamam Ul Haque
- Olga S Ovchinnikova
- Paul Abraham
- Radu Custelcean
- Ramanan Sankaran
- Serena Chen
- Udaya C Kalluri
- Vilmos Kertesz
- Vimal Ramanuj
- Vincent Paquit
- Wenjun Ge
- Yang Liu
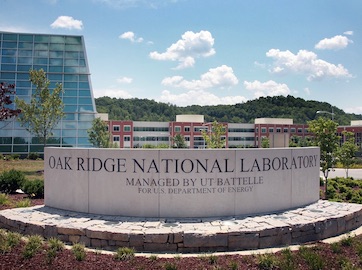
ORNL has developed bacterial strains that can utilize a common plastic co-monomer as a feedstock. This will help enable modern, petroleum-derived plastics to be converted into value-added chemicals.
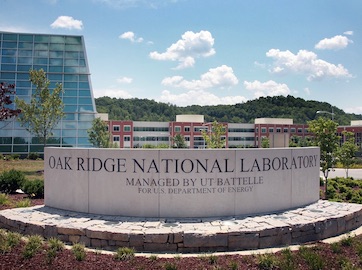
We have developed bacterial strains that can convert sustainable feedstocks and waste feedstocks into chemical precursors for next generation plastics.
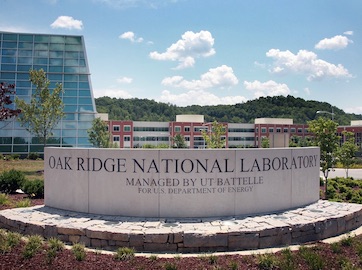
ORNL has identified a panel of novel nylon hydrolases with varied substrate and product selectivity.
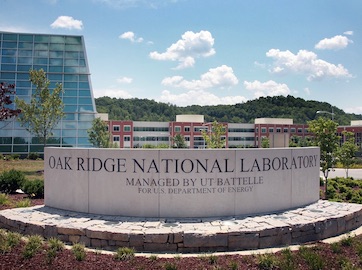
Genetic modification of microbes that are thermophiles—ones that grow at elevated temperatures—is extremely challenging. Tools developed for E. coli, a typical host for protein production, typically do not function at elevated temperatures.
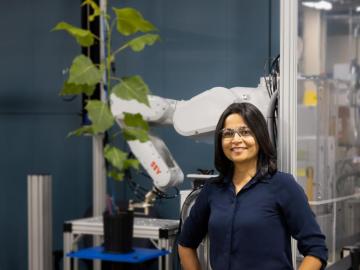
An innovative system for automating the surveillance and manipulation of plant tissues using advanced machine vision and robotic tools.

This innovative approach combines optical and spectral imaging data via machine learning to accurately predict cancer labels directly from tissue images.