Topics:
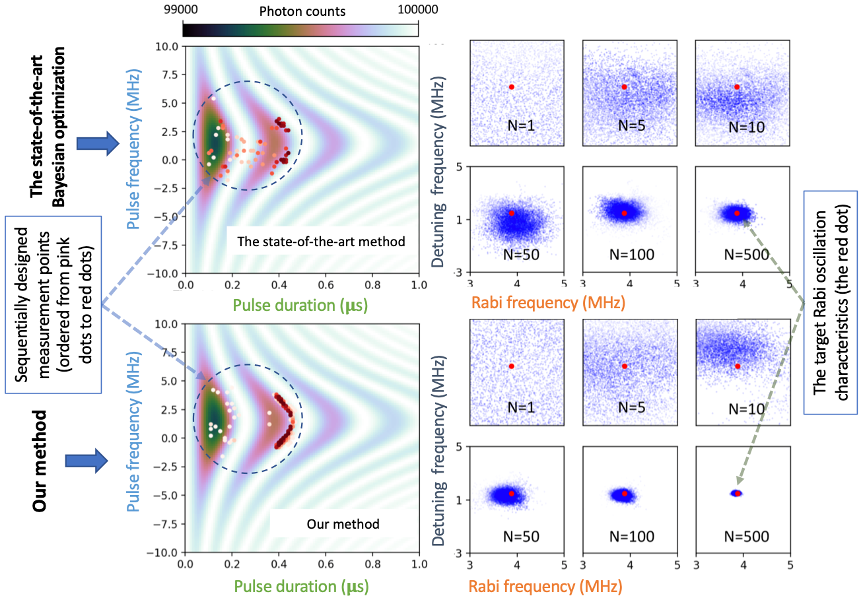
Radiofrequency pulse is an approach to control Rabi oscillation for quantum optics, MRI, etc. The ORNL method enables the ideal location of photon measurements in the pulse frequency and duration space to achieve a Rabi oscillation with desired Rabi and detuning frequencies. Left: the designed measurement points (from pink dots to red dots). Right: the evolution of the posterior distribution (the blue clouds) with the increase of measurement from 1 to 500.
The Science
ORNL researchers developed a stochastic approximate gradient ascent method to reduce posterior uncertainty in Bayesian experimental design involving implicit models.
This method:
- introduces a neural-network-based mutual information estimator into the objective function to replace the computationally intractable likelihood function, and
- can simultaneously train the mutual information estimator and search for an optimal design.
The Impact
This method:
- achieves designs with significantly improved confidence, i.e., small uncertainty of the posterior distribution when compared to the state-of-the-art Bayesian optimization (BO) method, and
- avoids intractable overhead cost of BO in solving high-dimensional problems.
PI: Guannan Zhang
Publication: J. Zhang, S. Bi, and G. Zhang, A hybrid gradient method to designing Bayesian experiments for implicit models, NeurIPS Workshop Proceeding on Machine Learning and the Physical Sciences, Dec. 2020. (PDF file).
ASCR Program/Facility: DOE ASCR Applied Math
Funding: DOE ASCR Applied Math (UQ4ML), and the ORNL AI Initiative
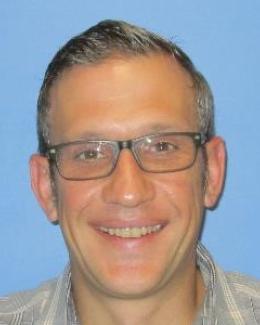