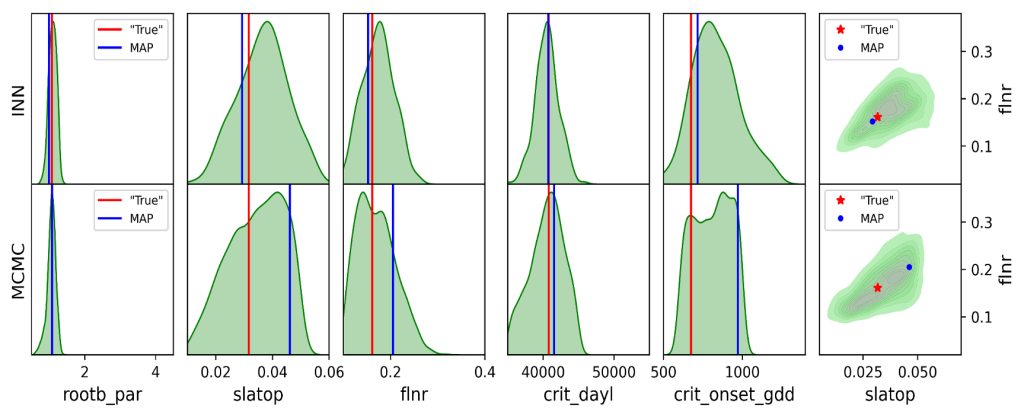
The Science
Oak Ridge National Laboratory researchers developed an invertible neural network (INN) to effectively and efficiently solve earth-system model calibration and simulation problems. The INN provides bijective mapping between model inputs and outputs to solve probabilistic inverse problems and forward approximations simultaneously. The INN consists of a sequence of reversible blocks developed from a normalizing flow strategy and trained using paired samples of model inputs and outputs. The trained network produces parameter posterior distributions by inverse evaluation for a given observation and generates corresponding model outputs by forward evaluation for a given parameter sample.
The Impact
The proposed INN has the potential to fundamentally change how model calibration and simulation are made in traditional earth system modeling. The INN is computationally efficient, solving inverse and forward problems in seconds after training, and thus can be used for accurate parameter estimation and prediction in scenarios where rapid evaluations are required.
PI/Facility Lead: Dan Lu
Funding: DnC2S project funded by DOE-ASCR; TES-SFA project funded by DOE-BER and the LDRD AI Initiative
Publication:
- Lu, D., Ricciuto, D., and Zhang J., “Invertible neural networks for E3SM land model calibration and simulation.” Accepted in ICLR Conference AI for Earth and Space Sciences Topic. Source: https://ai4earthscience.github.io/iclr-2022-workshop/accepted
Summary
We apply an invertible neural network (INN) for E3SM land model calibration and simulation with eight parameters at the Missouri Ozark AmeriFlux forest site. INN provides bijective (two-way) mappings between inputs and outputs, thus it can solve probabilistic inverse problems and forward approximations simultaneously. We demonstrate INN's inverse and forward capability in both synthetic and real-data applications. Results indicate that INN produces accurate parameter posterior distributions similar to Markov Chain Monte Carlo sampling and it generates model outputs close to the forward model simulations. Additionally, both the inverse and forward evaluations in INN are computationally efficient which allows for rapid integration of observations for parameter estimation and fast model predictions.