Filter News
Area of Research
- (-) Building Technologies (3)
- (-) Supercomputing (43)
- (-) Transportation Systems (2)
- Advanced Manufacturing (1)
- Biology and Environment (14)
- Clean Energy (105)
- Computational Biology (1)
- Computational Engineering (1)
- Computer Science (7)
- Electricity and Smart Grid (1)
- Functional Materials for Energy (1)
- Fusion and Fission (5)
- Isotopes (24)
- Materials (38)
- Materials for Computing (6)
- National Security (15)
- Neutron Science (11)
- Nuclear Science and Technology (5)
News Topics
- (-) Artificial Intelligence (36)
- (-) Buildings (7)
- (-) Isotopes (1)
- (-) Transportation (8)
- 3-D Printing/Advanced Manufacturing (6)
- Advanced Reactors (1)
- Big Data (19)
- Bioenergy (9)
- Biology (11)
- Biomedical (17)
- Biotechnology (2)
- Chemical Sciences (5)
- Climate Change (17)
- Computer Science (96)
- Coronavirus (14)
- Critical Materials (3)
- Cybersecurity (8)
- Decarbonization (6)
- Energy Storage (8)
- Environment (21)
- Exascale Computing (22)
- Frontier (28)
- Fusion (1)
- Grid (5)
- High-Performance Computing (38)
- Machine Learning (14)
- Materials (15)
- Materials Science (17)
- Mathematics (1)
- Microscopy (7)
- Molten Salt (1)
- Nanotechnology (11)
- National Security (8)
- Net Zero (1)
- Neutron Science (13)
- Nuclear Energy (4)
- Partnerships (1)
- Physics (7)
- Polymers (2)
- Quantum Computing (19)
- Quantum Science (24)
- Security (5)
- Simulation (14)
- Software (1)
- Space Exploration (3)
- Summit (42)
- Sustainable Energy (12)
Media Contacts

Researchers at ORNL are teaching microscopes to drive discoveries with an intuitive algorithm, developed at the lab’s Center for Nanophase Materials Sciences, that could guide breakthroughs in new materials for energy technologies, sensing and computing.
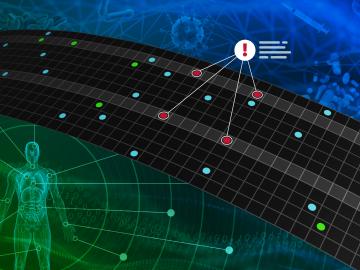
A team of researchers has developed a novel, machine learning–based technique to explore and identify relationships among medical concepts using electronic health record data across multiple healthcare providers.

A study led by researchers at ORNL could help make materials design as customizable as point-and-click.
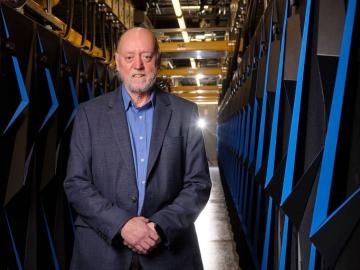
A force within the supercomputing community, Jack Dongarra developed software packages that became standard in the industry, allowing high-performance computers to become increasingly more powerful in recent decades.
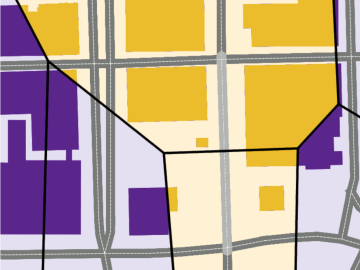
Every day, hundreds of thousands of commuters across the country travel from houses, apartments and other residential spaces to commercial buildings — from offices and schools to gyms and grocery stores.

A team of scientists led by the Department of Energy’s Oak Ridge National Laboratory and the Georgia Institute of Technology is using supercomputing and revolutionary deep learning tools to predict the structures and roles of thousands of proteins with unknown functions.
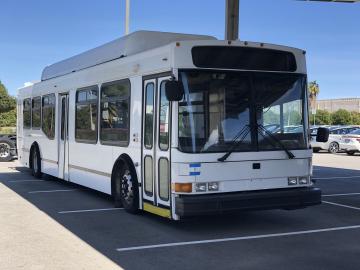
Oak Ridge National Laboratory researchers developed and demonstrated algorithm-based controls for a hybrid electric bus that yielded up to 30% energy savings compared with existing controls.

The daily traffic congestion along the streets and interstate lanes of Chattanooga could be headed the way of the horse and buggy with help from ORNL researchers.

Oak Ridge National Laboratory researchers designed and field-tested an algorithm that could help homeowners maintain comfortable temperatures year-round while minimizing utility costs.

An ORNL-led team comprising researchers from multiple DOE national laboratories is using artificial intelligence and computational screening techniques – in combination with experimental validation – to identify and design five promising drug therapy approaches to target the SARS-CoV-2 virus.