Filter News
Area of Research
News Topics
- (-) Coronavirus (3)
- (-) Environment (3)
- (-) Exascale Computing (2)
- (-) Machine Learning (2)
- 3-D Printing/Advanced Manufacturing (2)
- Artificial Intelligence (3)
- Big Data (1)
- Bioenergy (3)
- Biology (4)
- Biomedical (4)
- Buildings (1)
- Chemical Sciences (2)
- Clean Water (1)
- Climate Change (3)
- Composites (2)
- Computer Science (6)
- Critical Materials (3)
- Decarbonization (2)
- Energy Storage (1)
- Frontier (3)
- High-Performance Computing (5)
- Isotopes (2)
- Materials (14)
- Materials Science (4)
- Microscopy (3)
- Nanotechnology (4)
- National Security (1)
- Neutron Science (2)
- Physics (1)
- Polymers (1)
- Quantum Computing (5)
- Quantum Science (4)
- Simulation (4)
- Summit (5)
- Sustainable Energy (1)
Media Contacts
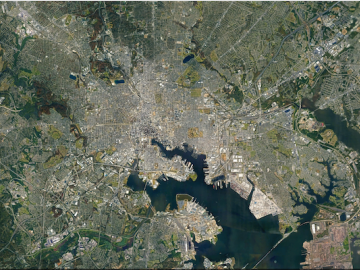
ORNL researchers are deploying their broad expertise in climate data and modeling to create science-based mitigation strategies for cities stressed by climate change as part of two U.S. Department of Energy Urban Integrated Field Laboratory projects.

A new paper published in Nature Communications adds further evidence to the bradykinin storm theory of COVID-19’s viral pathogenesis — a theory that was posited two years ago by a team of researchers at the Department of Energy’s Oak Ridge National Laboratory.
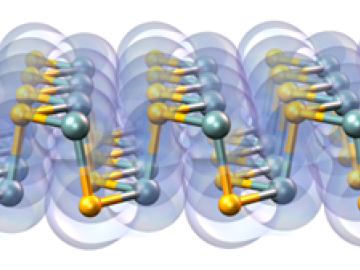
A multi-lab research team led by ORNL's Paul Kent is developing a computer application called QMCPACK to enable precise and reliable predictions of the fundamental properties of materials critical in energy research.
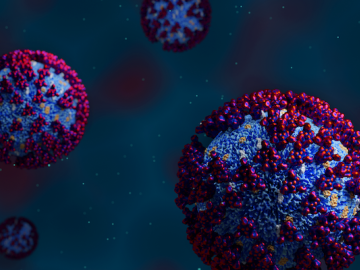
Researchers from ORNL, the University of Tennessee at Chattanooga and Tuskegee University used mathematics to predict which areas of the SARS-CoV-2 spike protein are most likely to mutate.
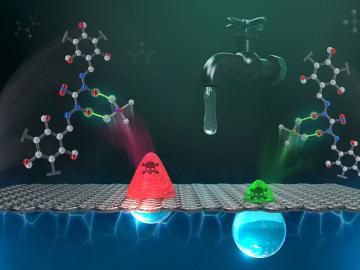
Researchers at ORNL are tackling a global water challenge with a unique material designed to target not one, but two toxic, heavy metal pollutants for simultaneous removal.
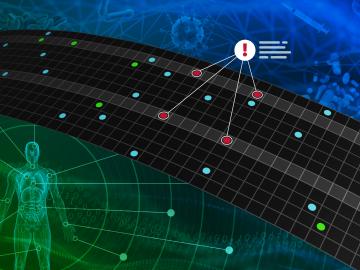
A team of researchers has developed a novel, machine learning–based technique to explore and identify relationships among medical concepts using electronic health record data across multiple healthcare providers.

To explore the inner workings of severe acute respiratory syndrome coronavirus 2, or SARS-CoV-2, researchers from ORNL developed a novel technique.

A new version of the Energy Exascale Earth System Model, or E3SM, is two times faster than an earlier version released in 2018.

A team of scientists led by the Department of Energy’s Oak Ridge National Laboratory and the Georgia Institute of Technology is using supercomputing and revolutionary deep learning tools to predict the structures and roles of thousands of proteins with unknown functions.