Project Details
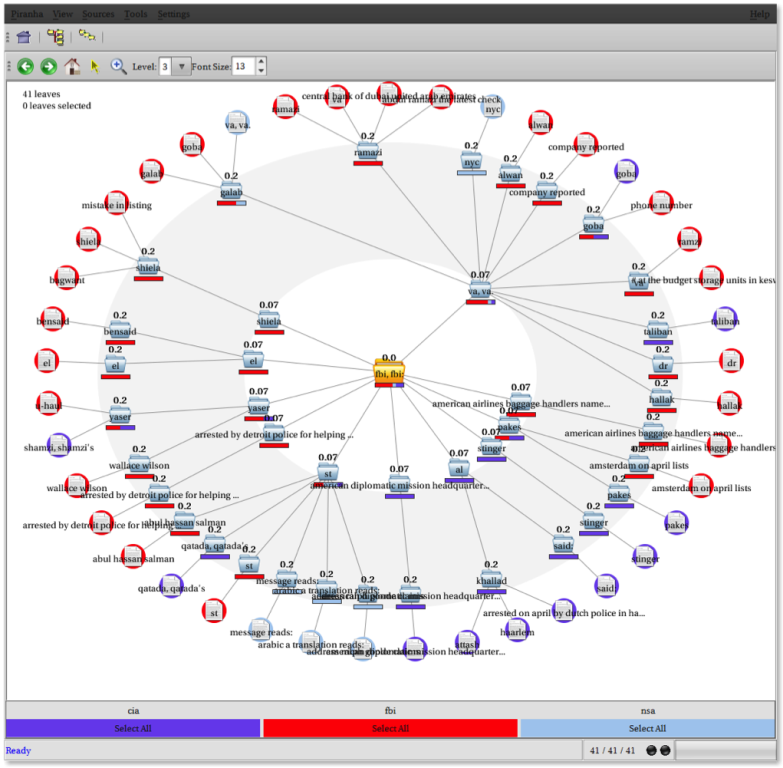
Big data demands the need for intelligent, recommender agents that can enhance a person’s situational or domain awareness of their environment. The ability to have a keen awareness and availability of relevant information provides a critical competitive edge. Unfortunately, there is simply too much data streaming too quickly for a person to manually process, analyze, and take action within a reasonable amount of time. In an attempt to alleviate this challenge, many people subscribe to relevant Internet information. There may be forms of subscriptions with the most common being Really Simple Syndication (RSS), blogs, even Facebook and Twitter. The concept is simple, when new information is posted to the site; a subscriber sees a list of this new information. The subscriber then has the option of following a link to read more. This approach is a very useful and successful model for monitoring this data, but it does have some significant drawbacks. In practice, the feeds of new information become quite lengthy, and contain more information than can be practically read. Furthermore, there can be a significant number of items that have little interest to the subscriber. Thus, the ability to find new and relevant information proves critical. We have developed a content-based recommender system that addresses both of these problems. The flexibility of input allows the system to be adaptable to industry and government use cases and data sets such as news feeds, resumes, proposal requests, etc.
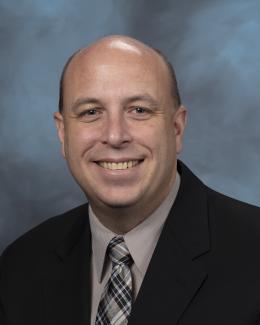