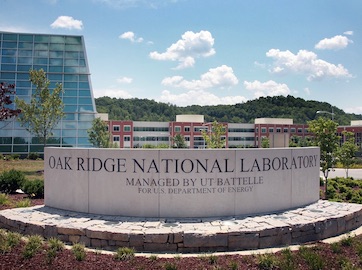
A multidisciplinary team of researchers from Oak Ridge National Laboratory (ORNL) pioneered the use of the LLVM-based high-productivity/high-performance Julia language unifying capabilities to write an end-to-end workflow on Frontier, the first US Depar