
Achievement: A multidisciplinary team of researchers from Oak Ridge National Laboratory (ORNL) and other institutions created a Machine Learning (ML) library for the training of classifiers on spectrographic chemical data. These classification models can then be used to help in the identification of unknown samples by classifying them according to their own spectrographic data. The Compendium of Uranium, Raman, and Infrared Experimental Specta (CURIES) dataset was ingested into a database in order to form the training data. Models were then trained to identify a variety of features such as secondary chemistry, structure type, or the coordination polyhedron.
Significance and Impact: Identification of unknown uranium chemistry samples is an important but challenging problem. Previous work in similar fields has focused on sample matching in order to find the most similar known mineral. The innovation of the Smart Spectral Matching (SSM) library is identify sample qualities piecemeal by training models to identify discrete qualities so that the unknown sample can be narrowed down in each category to aid in identification. This has produced a secondary knowledge gain in that the trained models themselves can reveal previously unknown features of classes of physical qualities for uranium minerals. The potential uses include the identification of unknown uranium samples, the discovery of novel features of uranium spectra, and the potential for expanding the set of trained models for the classification of other types of spectra, such as plutonium minerals.
Research Details
- A Machine Learning (ML) library was written for the identification of the qualities of unknown spectral sample data.
- The library was used to produce trained models for classification of uranium mineral samples based on secondary chemistry, structure type, and the coordination polyhedron.
- These trained models revealed new features of spectrographic data for sub-categories of uranium minerals.
PI and affiliation: Ashley Shields, Materials and Chemistry Group, Nuclear Non-proliferation Division, ORNL
Team: Ashley Shields, L. Drane, I. Gibbs, M. McDonnell, J. Niedziela, R. Smith, T. Spano (ORNL), S. Chalk (University of North Florida), T. Olds (Carnegie Museum of Natural History)
Summary: A ML library was written, designed to identify material characteristics given the sample’s spectrographic data. The library was used to train models with the CURIES dataset, resulting in a collection of classifiers. These classifiers can now be used to aid in the identification of unknown samples via identifying key chemical characteristics such as secondary chemistry, structure type, or the coordination polyhedron. In addition, the classifiers themselves have revealed previously unknown features of spectrographic data witch correlate with characteristics of uranium compounds.
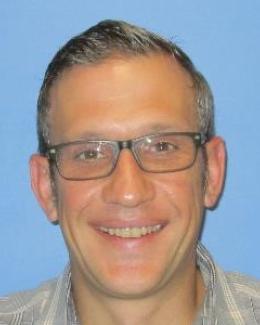