Scientific Achievement
We developed a novel uncertainty-aware framework MatPhase to predict material phases of electrodes from low contrast SEM images
Significance and Impact
- Matphase on average increase performance up to 8.5% against SOTA image segmentation models.
- MatPhase can provide an understanding to battery researchers about the material distribution of an electrode.
- MatPhase helps understanding battery degradation from cross- section of electrodes
Research Details
- We built a multi-class classifier to capture both local and global representations for hard data and aid in smoothening the phase boundaries.
- Introduce a hierarchical curriculum learning technique to predict material phase boundaries.
Citation and DOI:
- Tabassum, Anika, Nikhil Muralidhar, Ramakrishnan Kannan, and Srikanth Allu. "MatPhase: Material phase prediction for Li-ion Battery Reconstruction using Hierarchical Curriculum Learning." In 2022 IEEE Big Data, pp. 1936-1941.
- Tabassum, Anika, Nikhil Muralidhar, Ramakrishnan Kannan, and Srikanth Allu. "Li-ion Battery Material phase prediction through Hierarchical Curriculum Learning.” NeurIPS 2021 AI for Science Workshop.
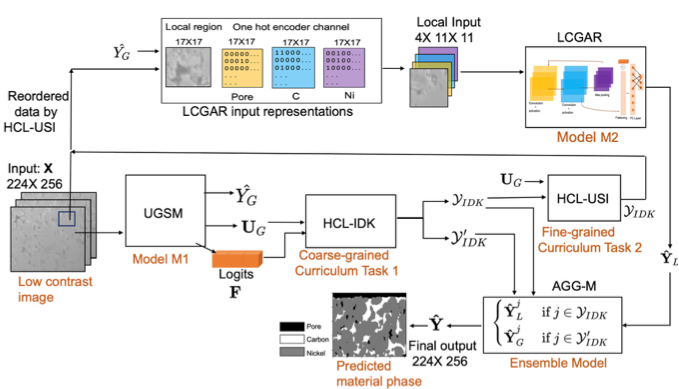
Summary:
Li-ion Batteries (LIB), one of the most efficient energy storage devices, are used extensively in many industrial applications. These batteries consist of electrodes that are put together with heterogeneous material compositions. Imaging data of these battery electrodes obtained from X-ray tomography can explain the distribution of material constituents and allow reconstructions to study electron transport pathways. Such reconstructions of material constituents help quantify various associated properties of electrodes (e.g., volume-specific surface area, porosity) which determine the performance of batteries. These images often suffer from low image contrast between multiple material constituents, hence making it difficult for humans to distinguish and characterize these constituents through visual inspection. A minor error in detecting distributions of the material constituents can lead to magnified errors in the calculated parameters of material properties (e.g., porosity). We present MatPhase, a novel hierarchical curriculum learning technique to address the complex task of estimating material constituent distribution in battery electrodes. MatPhase comprises three modules: (i) an uncertainty-aware global model trained to yield inferences conditioned upon global knowledge of material distribution, (ii) a local model to capture relatively more fine-grained (local) distributional signals, (iii) an aggregator model to appropriately fuse the local and global effects towards obtaining the final distribution. On average, MatPhase improves prediction up to 8.5% relative to other sophisticated modeling pipelines and state-of-the-arts (SOTA) object detection models employed in the performance comparison.
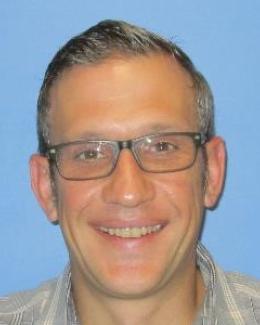