Invention Reference Number
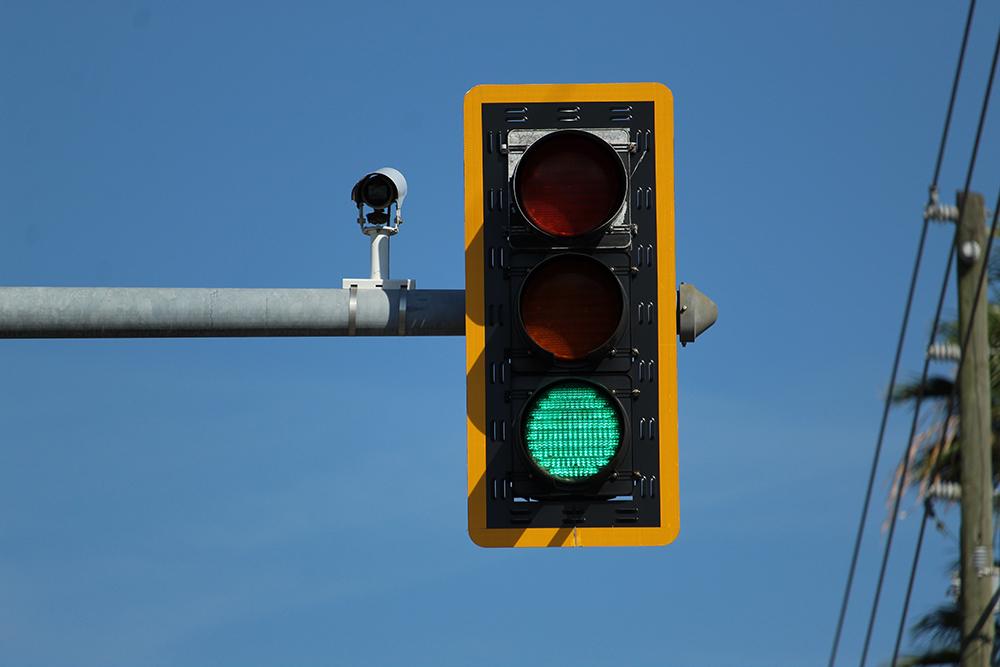
Pairing hybrid neural network modeling techniques with artificial intelligence, or AI, controls has resulted in a unique hybrid system that creates a smart solution for traffic-signal timing. Applied to multiple vehicle intersections along a single corridor, or across a broad range of traffic-signal layouts amid varying traffic conditions, this invention enables smoother traffic flow, resulting in reduced congestion and a reduction in the energy required to operate the system. Advanced dynamic modeling techniques provide this system with multiple data-rich measurements for analysis, including traffic demand and flow speed, signal timing, delays, queue length, and energy consumed over time, allowing for optimization of flow patterns and power usage.
Description
Artificial neural networks using AI modeling and controls for networked traffic systems are well documented. However, most studies have been limited to simulation-based analysis, due to a lack of comprehensive real-time data and user-friendly interfaces. Most related studies have also overlooked energy efficiency. Key factors in traffic buildup and congestion include traffic-signal timing, travel demand and delays, queue length, and number of stops. Applying AI and modeling technology for analysis can help facilitate the goal of coordinating signal control along congested routes by producing coordinated signal timing of multiple intersections simultaneously.
This invention is a result of a collaboration between the U.S. Department of Energy, Oak Ridge National Laboratory, the University of Hawaii, the Hawaiian Department of Transportation, and Econolite Systems. The project analyzed data from signal-control status parameters, closed circuit television-based video, vehicle-to-everything communication, and customized connect-vehicle trajectories, as well as real-time, advanced traveler-information systems. A closed-loop feedback system using a typical multi-objective stochastic optimization model allows AI to analyze and implement improved traffic guidance. Testing goals at the site in Hawaii targeted a 15% reduction in energy expenditure and a 25% reduction in travel delays. The solution obtained from this research is applicable to a wide range of traffic conditions.
Benefits
- Significant reduction of traffic congestion, delays, and energy use
- Enhanced mobility for drivers and improved traffic flow for municipalities
- Current compatibility with signal-control systems marketed by Econolite
Applications and Industries
- Linked traffic signals on single corridors and across larger applications
- Traffic signal manufacturers, vendors, and municipalities
Contact
To learn more about this technology, email partnerships@ornl.gov or call 865-574-1051.