Filter Results
Related Organization
- Biological and Environmental Systems Science Directorate (26)
- Computing and Computational Sciences Directorate (38)
- Energy Science and Technology Directorate (223)
- Fusion and Fission Energy and Science Directorate (24)
- Information Technology Services Directorate (3)
- Isotope Science and Enrichment Directorate (7)
- National Security Sciences Directorate (20)
- Neutron Sciences Directorate (11)
- Physical Sciences Directorate (135)
- User Facilities
(27)
Researcher
- Kyle Kelley
- Rama K Vasudevan
- Olga S Ovchinnikova
- Sergei V Kalinin
- Alexandre Sorokine
- Anton Ievlev
- Bogdan Dryzhakov
- Clinton Stipek
- Daniel Adams
- Debangshu Mukherjee
- Jessica Moehl
- Josh Michener
- Kevin M Roccapriore
- Liam Collins
- Liangyu Qian
- Marti Checa Nualart
- Maxim A Ziatdinov
- Md Inzamam Ul Haque
- Neus Domingo Marimon
- Philipe Ambrozio Dias
- Serena Chen
- Stephen Jesse
- Steven Randolph
- Taylor Hauser
- Viswadeep Lebakula
- Yongtao Liu
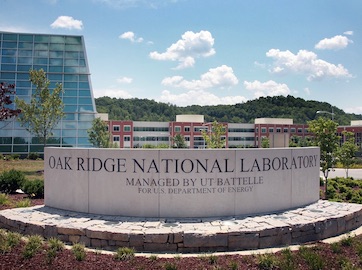
We tested 48 diverse homologs of SfaB and identified several enzyme variants that were more active than SfaB at synthesizing the nylon-6,6 monomer.
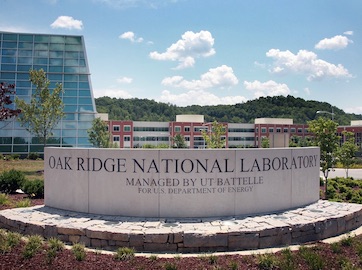
Understanding building height is imperative to the overall study of energy efficiency, population distribution, urban morphologies, emergency response, among others. Currently, existing approaches for modelling building height at scale are hindered by two pervasive issues.

The invention introduces a novel, customizable method to create, manipulate, and erase polar topological structures in ferroelectric materials using atomic force microscopy.

High coercive fields prevalent in wurtzite ferroelectrics present a significant challenge, as they hinder efficient polarization switching, which is essential for microelectronic applications.
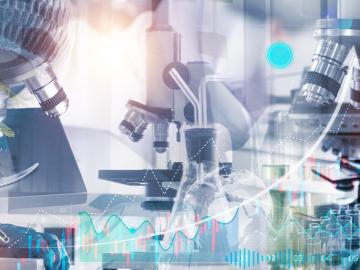
This invention presents technologies for characterizing physical properties of a sample's surface by combining image processing with machine learning techniques.

This invention introduces a system for microscopy called pan-sharpening, enabling the generation of images with both full-spatial and full-spectral resolution without needing to capture the entire dataset, significantly reducing data acquisition time.

This innovative approach combines optical and spectral imaging data via machine learning to accurately predict cancer labels directly from tissue images.