Filter Results
Related Organization
- Biological and Environmental Systems Science Directorate (23)
- Computing and Computational Sciences Directorate (35)
- Energy Science and Technology Directorate (217)
- Fusion and Fission Energy and Science Directorate (21)
- Information Technology Services Directorate (2)
- Isotope Science and Enrichment Directorate (6)
- National Security Sciences Directorate
(17)
- Neutron Sciences Directorate (11)
- Physical Sciences Directorate (128)
- User Facilities
(27)
Researcher
- Kyle Kelley
- Rama K Vasudevan
- Olga S Ovchinnikova
- Sergei V Kalinin
- Aaron Werth
- Alexander I Wiechert
- Ali Passian
- Anton Ievlev
- Bogdan Dryzhakov
- Costas Tsouris
- Debangshu Mukherjee
- Emilio Piesciorovsky
- Gary Hahn
- Gs Jung
- Gyoung Gug Jang
- Harper Jordan
- Joel Asiamah
- Joel Dawson
- Kevin M Roccapriore
- Liam Collins
- Marti Checa Nualart
- Maxim A Ziatdinov
- Md Inzamam Ul Haque
- Nance Ericson
- Neus Domingo Marimon
- Radu Custelcean
- Raymond Borges Hink
- Srikanth Yoginath
- Stephen Jesse
- Steven Randolph
- Varisara Tansakul
- Yarom Polsky
- Yongtao Liu
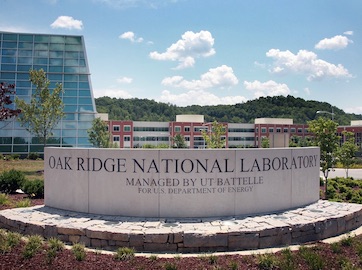
Among the methods for point source carbon capture, the absorption of CO2 using aqueous amines (namely MEA) from the post-combustion gas stream is currently considered the most promising.

The invention introduces a novel, customizable method to create, manipulate, and erase polar topological structures in ferroelectric materials using atomic force microscopy.

High coercive fields prevalent in wurtzite ferroelectrics present a significant challenge, as they hinder efficient polarization switching, which is essential for microelectronic applications.

Electrical utility substations are wired with intelligent electronic devices (IEDs), such as protective relays, power meters, and communication switches.
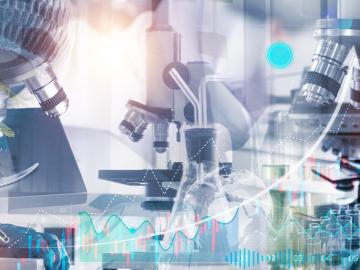
This invention presents technologies for characterizing physical properties of a sample's surface by combining image processing with machine learning techniques.

This invention introduces a system for microscopy called pan-sharpening, enabling the generation of images with both full-spatial and full-spectral resolution without needing to capture the entire dataset, significantly reducing data acquisition time.

This innovative approach combines optical and spectral imaging data via machine learning to accurately predict cancer labels directly from tissue images.