Filter Research Highlights
Area of Research

ORNL researchers developed a novel nonlinear level set learning method to reduce dimensionality in high-dimensional function approximation.
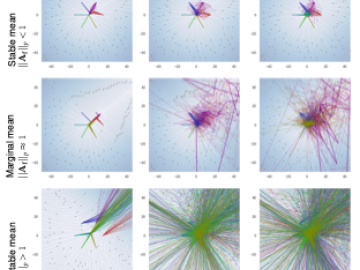
The team conducted numerical studies to demonstrate the connection between the parameters of neural networks and the stochastic stability of DMMs.
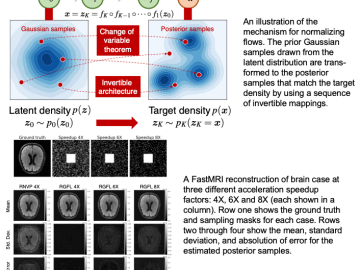
A research team from ORNL and Pacific Northwest National Laboratory has developed a deep variational framework to learn an approximate posterior for uncertainty quantification.

Estimating complex, non-linear model states and parameters from uncertain systems of equations and noisy observation data with current filtering methods is a key challenge in mathematical modeling.
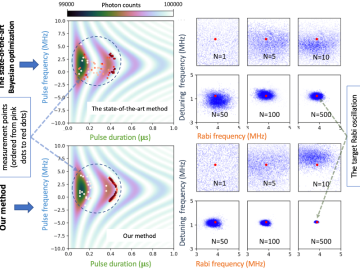
ORNL researchers developed a stochastic approximate gradient ascent method to reduce posterior uncertainty in Bayesian experimental design involving implicit models.
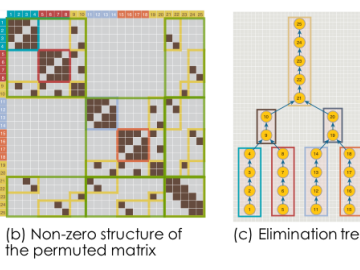
The researchers from ORNL have developed a new and faster algorithm for the graph all-pair shortest-path (APSP) problem.
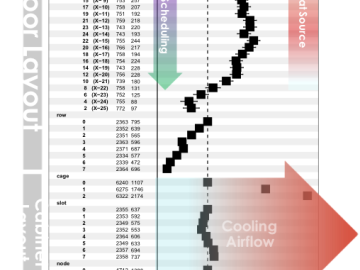
A team of researchers from Oak Ridge National Laboratory applied advanced statistical methods from biomedical research to study an unexpected failure mode of general-purpose computing on graphics processing units (GPGPUs).