Brief: Deep learning models have trained to predict crystallographic and thermodynamic properties of multi-component solid solution alloys, enabling the design of advanced alloys.
Accomplishment: Surrogate deep learning (DL) models were trained to predict crystallographic properties (e.g., lattice parameters) and thermodynamic properties (e.g., Gibbs free energy) of multi-component solid solution alloys as a function of element composition and temperature. The demonstrated approach will allow material scientists to efficiently leverage sparse data defined in a high dimensional space, thus overcoming the challenges of data scarcity in material science.
We developed a surrogate DL model trained on a dataset generated by the computational thermodynamics approach CALculation of PHAse Diagrams (CALPHAD). Using unary and binary data for crystallographic and thermodynamic quantities of interest (QoI) such as the lattice parameters and the Gibbs free energy, respectively, we trained the DL model to predict the same QoI for ternary systems.
Numerical results show that our DL model can extrapolate information about the lattice parameter from unary and binary elements to produce accurate predictions of the lattice parameters of ternary alloys thereby reducing the relative error with respect to more standard machine learning models from 15% to 0.1%. Moreover, these predictions are made in seconds as opposed to hour-long experiments.
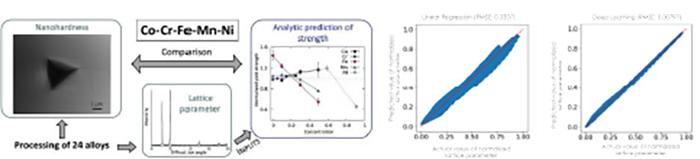
Acknowledgement: This research is funded by the AI Initiative, as part of the Laboratory Directed Research and Development Program of Oak Ridge National Laboratory, managed by UT-Battelle, LLC, for the U.S. Department of Energy (DOE).
Publications and presentations resulting from this work:
- Laiu, P., Shin, D., Yang, Y., Choi, J., Lupo Pasini, M., Reeve, S., Predicting Multicomponent Alloy Properties with Neural Network Surrogate Models, Presentation to the TMS World Congress on Artificial Intelligence in Materials and Manufacturing (AIM 2022) - Algorithm Development in Materials Science and Engineering mini-symposium.
- Lupo Pasini, M., Deep learning for prediction of material properties of solid solution alloys from multiscale information, ORNL Computational Mechanics Seminar Series, https://computmech.ornl.gov/seminars.html, February 24, 2022.
Contact: Massimiliano Lupo Pasini (lupopasinim@ornl.gov)
Team: Massimiliano (Max) Lupo Pasini, Paul Laiu, Dongwon Shin, Ying Yang, Jong Youl Choi, Samuel Temple Reeve
References:
- B. Sundman, H.L. Lukas, & S. G. Fries. (2007). Computational thermodynamics: the Calphad method. New York: Cambridge university press.
- S. Gorsse and O.N. Senkov, About the Reliability of CALPHAD Predictions in Multicomponent Systems. Entropy, 2018. 20(12): p. 899.