Filter News
Area of Research
- Advanced Manufacturing (2)
- Biology and Environment (14)
- Clean Energy (17)
- Computational Engineering (1)
- Computer Science (4)
- Electricity and Smart Grid (1)
- Energy Frontier Research Centers (1)
- Functional Materials for Energy (1)
- Fusion and Fission (2)
- Isotope Development and Production (1)
- Isotopes (4)
- Materials (43)
- Materials for Computing (8)
- National Security (12)
- Neutron Science (16)
- Nuclear Science and Technology (5)
- Quantum information Science (1)
- Supercomputing (25)
News Topics
- (-) Machine Learning (47)
- (-) Nanotechnology (60)
- (-) Space Exploration (25)
- 3-D Printing/Advanced Manufacturing (119)
- Advanced Reactors (34)
- Artificial Intelligence (91)
- Big Data (53)
- Bioenergy (91)
- Biology (98)
- Biomedical (58)
- Biotechnology (22)
- Buildings (57)
- Chemical Sciences (63)
- Clean Water (29)
- Climate Change (99)
- Composites (26)
- Computer Science (186)
- Coronavirus (46)
- Critical Materials (25)
- Cybersecurity (35)
- Decarbonization (78)
- Education (4)
- Element Discovery (1)
- Emergency (2)
- Energy Storage (108)
- Environment (194)
- Exascale Computing (37)
- Fossil Energy (5)
- Frontier (42)
- Fusion (53)
- Grid (62)
- High-Performance Computing (84)
- Hydropower (11)
- Irradiation (3)
- Isotopes (52)
- ITER (7)
- Materials (143)
- Materials Science (138)
- Mathematics (7)
- Mercury (12)
- Microelectronics (2)
- Microscopy (51)
- Molten Salt (8)
- National Security (60)
- Net Zero (13)
- Neutron Science (130)
- Nuclear Energy (106)
- Partnerships (42)
- Physics (59)
- Polymers (33)
- Quantum Computing (32)
- Quantum Science (67)
- Renewable Energy (2)
- Security (24)
- Simulation (46)
- Software (1)
- Statistics (3)
- Summit (57)
- Sustainable Energy (125)
- Transformational Challenge Reactor (7)
- Transportation (95)
Media Contacts
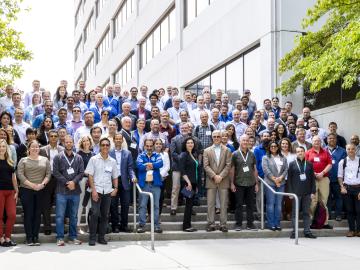
ORNL hosted its annual Smoky Mountains Computational Sciences and Engineering Conference in person for the first time since the COVID-19 pandemic.
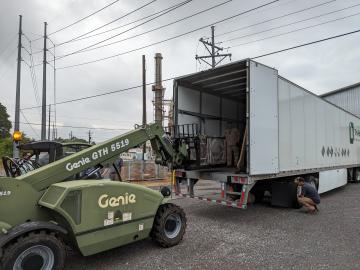
In June, ORNL hit a milestone not seen in more than three decades: producing a production-quality amount of plutonium-238

Speakers, scientific workshops, speed networking, a student poster showcase and more energized the Annual User Meeting of the Department of Energy’s Center for Nanophase Materials Sciences, or CNMS, Aug. 7-10, near Market Square in downtown Knoxville, Tennessee.
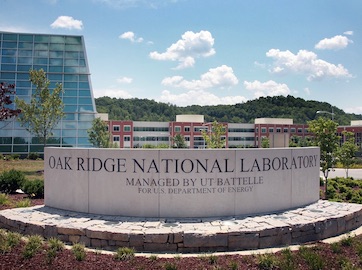
The Department of Energy’s Office of Science has selected three ORNL research teams to receive funding through DOE’s new Biopreparedness Research Virtual Environment initiative.
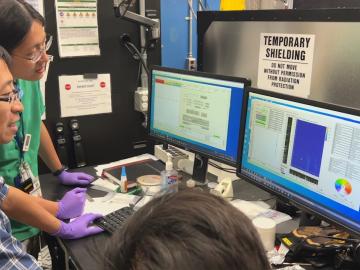
Neutron experiments can take days to complete, requiring researchers to work long shifts to monitor progress and make necessary adjustments. But thanks to advances in artificial intelligence and machine learning, experiments can now be done remotely and in half the time.
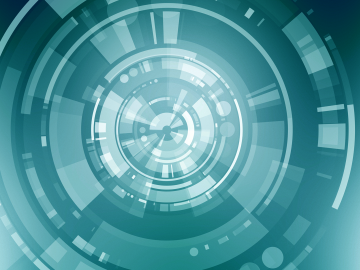
A new nanoscience study led by a researcher at ORNL takes a big-picture look at how scientists study materials at the smallest scales.

A licensing agreement between the Department of Energy’s Oak Ridge National Laboratory and research partner ZEISS will enable industrial X-ray computed tomography, or CT, to perform rapid evaluations of 3D-printed components using ORNL’s machine
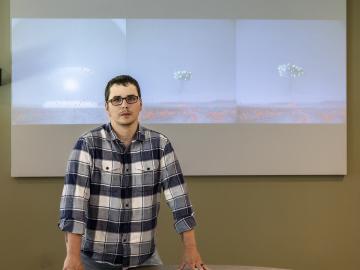
Cody Lloyd became a nuclear engineer because of his interest in the Manhattan Project, the United States’ mission to advance nuclear science to end World War II. As a research associate in nuclear forensics at ORNL, Lloyd now teaches computers to interpret data from imagery of nuclear weapons tests from the 1950s and early 1960s, bringing his childhood fascination into his career
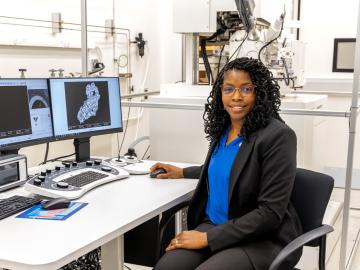
After completing a bachelor’s degree in biology, Toya Beiswenger didn’t intend to go into forensics. But almost two decades later, the nuclear security scientist at ORNL has found a way to appreciate the art of nuclear forensics.
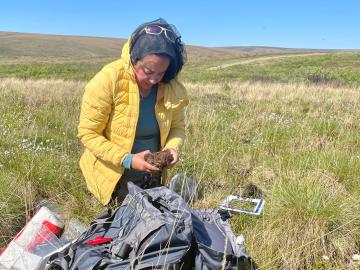
Wildfires are an ancient force shaping the environment, but they have grown in frequency, range and intensity in response to a changing climate. At ORNL, scientists are working on several fronts to better understand and predict these events and what they mean for the carbon cycle and biodiversity.