Filter News
Area of Research
News Topics
- (-) Clean Water (13)
- (-) Critical Materials (11)
- (-) Fusion (9)
- (-) Machine Learning (10)
- (-) Molten Salt (5)
- 3-D Printing/Advanced Manufacturing (30)
- Advanced Reactors (13)
- Artificial Intelligence (13)
- Big Data (15)
- Bioenergy (15)
- Biology (17)
- Biomedical (11)
- Biotechnology (3)
- Buildings (17)
- Chemical Sciences (7)
- Climate Change (20)
- Composites (9)
- Computer Science (39)
- Coronavirus (11)
- Cybersecurity (3)
- Decarbonization (7)
- Energy Storage (30)
- Environment (43)
- Exascale Computing (1)
- Frontier (1)
- Grid (20)
- High-Performance Computing (11)
- Hydropower (6)
- Irradiation (2)
- Isotopes (5)
- ITER (3)
- Materials (34)
- Materials Science (31)
- Mathematics (1)
- Mercury (3)
- Microscopy (11)
- Nanotechnology (12)
- National Security (3)
- Net Zero (1)
- Neutron Science (27)
- Nuclear Energy (18)
- Partnerships (1)
- Physics (4)
- Polymers (8)
- Quantum Computing (4)
- Quantum Science (10)
- Security (1)
- Simulation (6)
- Space Exploration (10)
- Statistics (1)
- Summit (6)
- Sustainable Energy (42)
- Transportation (35)
Media Contacts
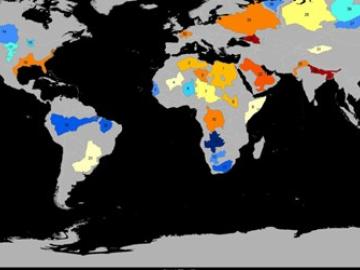
Groundwater withdrawals are expected to peak in about one-third of the world’s basins by 2050, potentially triggering significant trade and agriculture shifts, a new analysis finds.
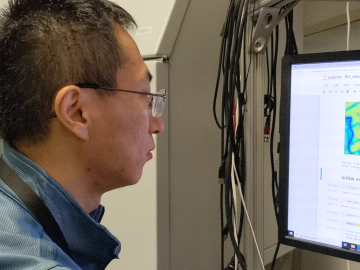
To capitalize on AI and researcher strengths, scientists developed a human-AI collaboration recommender system for improved experimentation performance.
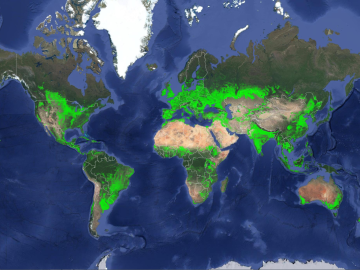
ORNL climate modeling expertise contributed to a project that assessed global emissions of ammonia from croplands now and in a warmer future, while also identifying solutions tuned to local growing conditions.
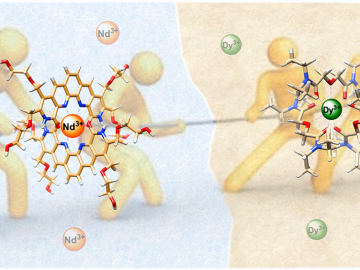
ORNL scientists combined two ligands, or metal-binding molecules, to target light and heavy lanthanides simultaneously for exceptionally efficient separation.
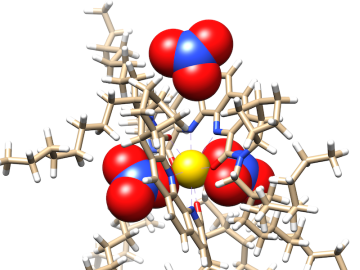
Researchers at ORNL zoomed in on molecules designed to recover critical materials via liquid-liquid extraction — a method used by industry to separate chemically similar elements.

Critical Materials Institute researchers at Oak Ridge National Laboratory and Arizona State University studied the mineral monazite, an important source of rare-earth elements, to enhance methods of recovering critical materials for energy, defense and manufacturing applications.
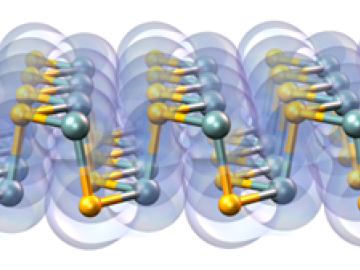
A multi-lab research team led by ORNL's Paul Kent is developing a computer application called QMCPACK to enable precise and reliable predictions of the fundamental properties of materials critical in energy research.

Researchers at Oak Ridge National Laboratory and Momentum Technologies have piloted an industrial-scale process for recycling valuable materials in the millions of tons of e-waste generated annually in the United States.

Researchers at Oak Ridge National Laboratory are using state-of-the-art methods to shed light on chemical separations needed to recover rare-earth elements and secure critical materials for clean energy technologies.
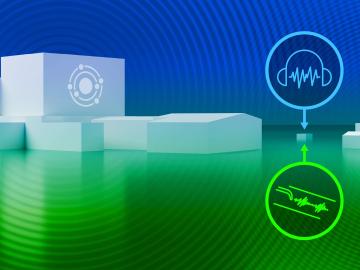
An Oak Ridge National Laboratory team developed a novel technique using sensors to monitor seismic and acoustic activity and machine learning to differentiate operational activities at facilities from “noise” in the recorded data.