
Filter News
Area of Research
- (-) Biology and Environment (11)
- (-) Supercomputing (29)
- Advanced Manufacturing (3)
- Clean Energy (37)
- Computational Biology (1)
- Computational Engineering (1)
- Computer Science (7)
- Electricity and Smart Grid (2)
- Fusion and Fission (5)
- Fusion Energy (6)
- Materials (22)
- Materials for Computing (3)
- National Security (19)
- Neutron Science (59)
- Nuclear Science and Technology (13)
- Nuclear Systems Modeling, Simulation and Validation (1)
- Quantum information Science (1)
- Sensors and Controls (1)
News Type
News Topics
- (-) Advanced Reactors (1)
- (-) Artificial Intelligence (25)
- (-) Grid (3)
- (-) Machine Learning (11)
- (-) Neutron Science (6)
- 3-D Printing/Advanced Manufacturing (4)
- Big Data (22)
- Bioenergy (36)
- Biology (58)
- Biomedical (20)
- Biotechnology (9)
- Buildings (2)
- Chemical Sciences (5)
- Clean Water (11)
- Climate Change (40)
- Composites (2)
- Computer Science (68)
- Coronavirus (13)
- Critical Materials (3)
- Cybersecurity (2)
- Decarbonization (18)
- Energy Storage (3)
- Environment (83)
- Exascale Computing (15)
- Frontier (14)
- Fusion (1)
- High-Performance Computing (33)
- Hydropower (8)
- Materials (7)
- Materials Science (11)
- Mathematics (3)
- Mercury (7)
- Microscopy (8)
- Nanotechnology (8)
- National Security (4)
- Net Zero (2)
- Nuclear Energy (3)
- Physics (4)
- Polymers (3)
- Quantum Computing (14)
- Quantum Science (13)
- Renewable Energy (1)
- Security (1)
- Simulation (17)
- Software (1)
- Space Exploration (2)
- Summit (29)
- Sustainable Energy (27)
- Transportation (5)
Media Contacts
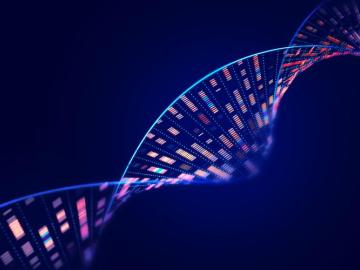
A team of researchers from ORNL was recognized by the National Cancer Institute in March for their unique contributions in the fight against cancer.
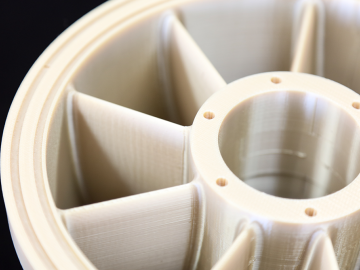
A new report published by ORNL assessed how advanced manufacturing and materials, such as 3D printing and novel component coatings, could offer solutions to modernize the existing fleet and design new approaches to hydropower.
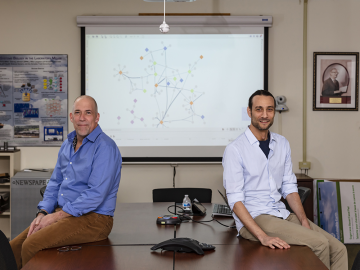
ORNL researchers discovered genetic mutations that underlie autism using a new approach that could lead to better diagnostics and drug therapies.
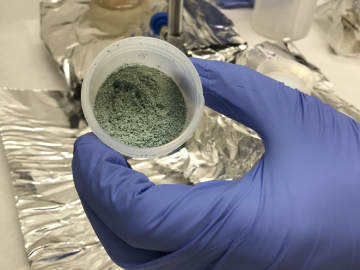
ORNL researchers used the nation’s fastest supercomputer to map the molecular vibrations of an important but little-studied uranium compound produced during the nuclear fuel cycle for results that could lead to a cleaner, safer world.
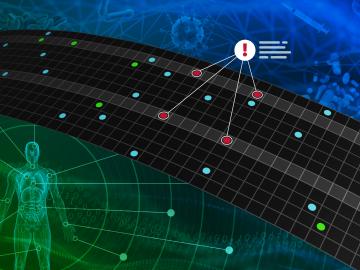
A team of researchers has developed a novel, machine learning–based technique to explore and identify relationships among medical concepts using electronic health record data across multiple healthcare providers.

A study led by researchers at ORNL could help make materials design as customizable as point-and-click.

ORNL scientists had a problem mapping the genomes of bacteria to better understand the origins of their physical traits and improve their function for bioenergy production.

Researchers at Oak Ridge National Laboratory are using a novel approach in determining environmental impacts to aquatic species near hydropower facilities, potentially leading to smarter facility designs that can support electrical grid reliability.
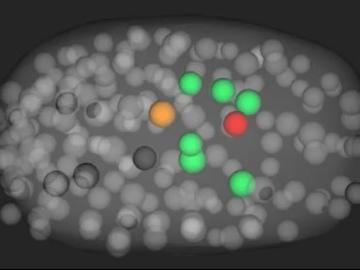
Scientists have developed a novel approach to computationally infer previously undetected behaviors within complex biological environments by analyzing live, time-lapsed images that show the positioning of embryonic cells in C. elegans, or roundworms. Their published methods could be used to reveal hidden biological activity.

A team of scientists led by the Department of Energy’s Oak Ridge National Laboratory and the Georgia Institute of Technology is using supercomputing and revolutionary deep learning tools to predict the structures and roles of thousands of proteins with unknown functions.