Filter News
Area of Research
News Type
News Topics
- (-) Advanced Reactors (9)
- (-) Clean Water (15)
- (-) Cybersecurity (14)
- (-) Isotopes (25)
- (-) Machine Learning (22)
- (-) Mathematics (5)
- (-) National Security (37)
- (-) Space Exploration (11)
- 3-D Printing/Advanced Manufacturing (38)
- Artificial Intelligence (44)
- Big Data (24)
- Bioenergy (49)
- Biology (57)
- Biomedical (29)
- Biotechnology (11)
- Buildings (23)
- Chemical Sciences (23)
- Climate Change (50)
- Composites (6)
- Computer Science (85)
- Coronavirus (18)
- Critical Materials (2)
- Decarbonization (48)
- Emergency (2)
- Energy Storage (34)
- Environment (106)
- Exascale Computing (24)
- Fossil Energy (4)
- Frontier (24)
- Fusion (31)
- Grid (25)
- High-Performance Computing (42)
- Hydropower (5)
- ITER (2)
- Materials (40)
- Materials Science (49)
- Mercury (7)
- Microelectronics (2)
- Microscopy (22)
- Molten Salt (1)
- Nanotechnology (20)
- Net Zero (8)
- Neutron Science (48)
- Nuclear Energy (58)
- Partnerships (13)
- Physics (31)
- Polymers (10)
- Quantum Computing (17)
- Quantum Science (28)
- Renewable Energy (1)
- Security (12)
- Simulation (29)
- Software (1)
- Summit (30)
- Sustainable Energy (45)
- Transformational Challenge Reactor (3)
- Transportation (32)
Media Contacts
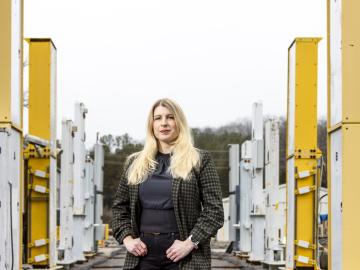
Researchers tackling national security challenges at ORNL are upholding an 80-year legacy of leadership in all things nuclear. Today, they’re developing the next generation of technologies that will help reduce global nuclear risk and enable safe, secure, peaceful use of nuclear materials, worldwide.
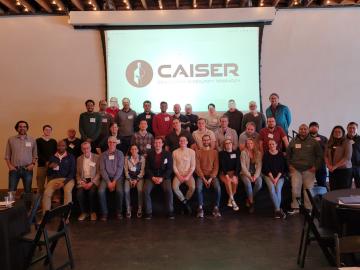
Researchers at the Department of Energy’s Oak Ridge National Laboratory met recently at an AI Summit to better understand threats surrounding artificial intelligence. The event was part of ORNL’s mission to shape the future of safe and secure AI systems charged with our nation’s most precious data.
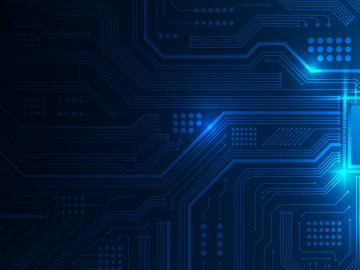
A team led by researchers at ORNL explored training strategies for one of the largest artificial intelligence models to date with help from the world’s fastest supercomputer. The findings could help guide training for a new generation of AI models for scientific research.

ORNL scientists are working on a project to engineer and develop a cryogenic ion trap apparatus to simulate quantum spin liquids, a key research area in materials science and neutron scattering studies.
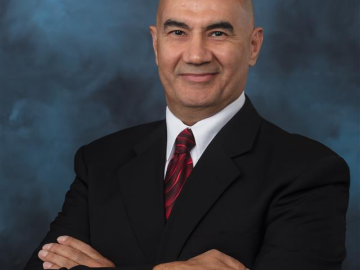
Mohamad Zineddin hopes to establish an interdisciplinary center of excellence for nuclear security at ORNL, combining critical infrastructure assessment and protection, risk mitigation, leadership in nuclear security, education and training, nuclear security culture and resilience strategies and techniques.

Researchers at ORNL are using a machine-learning model to answer ‘what if’ questions stemming from major events that impact large numbers of people. By simulating an event, such as extreme weather, researchers can see how people might respond to adverse situations, and those outcomes can be used to improve emergency planning.

To balance personal safety and research innovation, researchers at ORNL are employing a mathematical technique known as differential privacy to provide data privacy guarantees.

Scientists at Oak Ridge National Laboratory and six other Department of Energy national laboratories have developed a United States-based perspective for achieving net-zero carbon emissions.
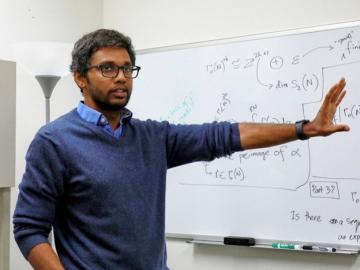
In the age of easy access to generative AI software, user can take steps to stay safe. Suhas Sreehari, an applied mathematician, identifies misconceptions of generative AI that could lead to unintentionally bad outcomes for a user.
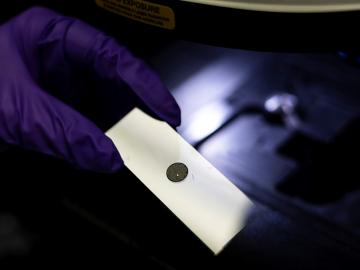
Nuclear nonproliferation scientists at ORNL have published the Compendium of Uranium Raman and Infrared Experimental Spectra, a public database and analysis of structure-spectral relationships for uranium minerals. This first-of-its-kind dataset and corresponding analysis fill a key gap in the existing body of knowledge for mineralogists and actinide scientists.