Filter News
Area of Research
News Topics
- (-) Machine Learning (6)
- (-) Nanotechnology (6)
- (-) Summit (1)
- 3-D Printing/Advanced Manufacturing (9)
- Advanced Reactors (2)
- Artificial Intelligence (8)
- Big Data (2)
- Bioenergy (6)
- Biology (6)
- Biomedical (3)
- Biotechnology (1)
- Buildings (13)
- Chemical Sciences (11)
- Clean Water (2)
- Climate Change (9)
- Composites (2)
- Computer Science (10)
- Coronavirus (4)
- Critical Materials (3)
- Cybersecurity (6)
- Decarbonization (14)
- Energy Storage (22)
- Environment (10)
- Exascale Computing (1)
- Fossil Energy (1)
- Frontier (2)
- Fusion (1)
- Grid (11)
- High-Performance Computing (5)
- Hydropower (1)
- Isotopes (2)
- Materials (26)
- Materials Science (9)
- Microscopy (5)
- National Security (13)
- Net Zero (1)
- Neutron Science (6)
- Nuclear Energy (2)
- Partnerships (6)
- Physics (5)
- Polymers (3)
- Quantum Science (2)
- Security (3)
- Simulation (2)
- Sustainable Energy (12)
- Transformational Challenge Reactor (1)
- Transportation (10)
Media Contacts
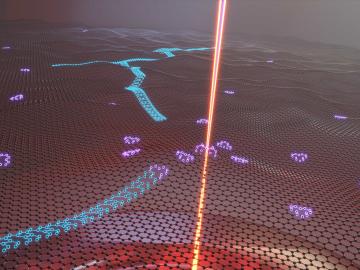
Oak Ridge National Laboratory researchers serendipitously discovered when they automated the beam of an electron microscope to precisely drill holes in the atomically thin lattice of graphene, the drilled holes closed up.
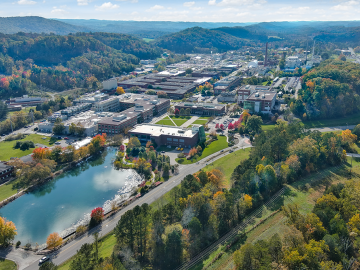
Eight ORNL scientists are among the world’s most highly cited researchers, according to a bibliometric analysis conducted by the scientific publication analytics firm Clarivate.
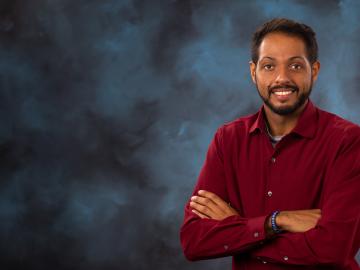
Having lived on three continents spanning the world’s four hemispheres, Philipe Ambrozio Dias understands the difficulties of moving to a new place.
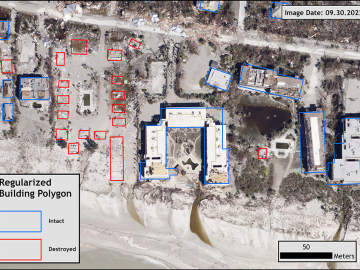
Over the past seven years, researchers in ORNL’s Geospatial Science and Human Security Division have mapped and characterized all structures within the United States and its territories to aid FEMA in its response to disasters. This dataset provides a consistent, nationwide accounting of the buildings where people reside and work.
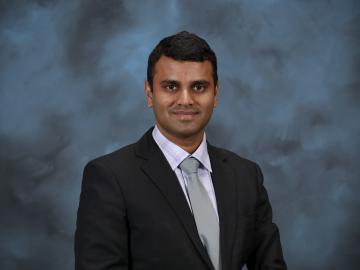
Rama Vasudevan, a research scientist at the Department of Energy’s Oak Ridge National Laboratory, has been elected a Fellow of the American Physical Society, or APS. The honor recognizes members who have made significant contributions to physics and its application to science and technology.
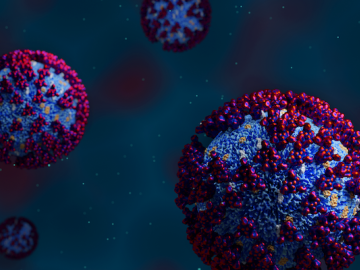
Researchers from ORNL, the University of Tennessee at Chattanooga and Tuskegee University used mathematics to predict which areas of the SARS-CoV-2 spike protein are most likely to mutate.
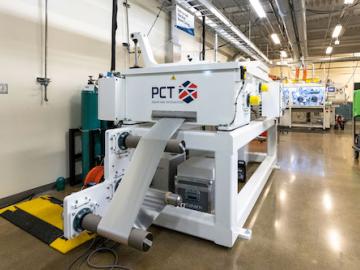
Researchers at the Department of Energy’s Oak Ridge National Laboratory and their technologies have received seven 2022 R&D 100 Awards, plus special recognition for a battery-related green technology product.

Researchers at ORNL are teaching microscopes to drive discoveries with an intuitive algorithm, developed at the lab’s Center for Nanophase Materials Sciences, that could guide breakthroughs in new materials for energy technologies, sensing and computing.
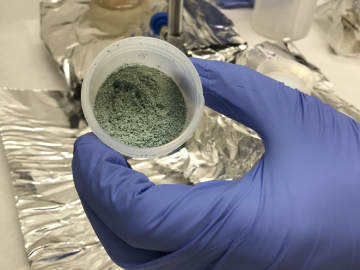
ORNL researchers used the nation’s fastest supercomputer to map the molecular vibrations of an important but little-studied uranium compound produced during the nuclear fuel cycle for results that could lead to a cleaner, safer world.
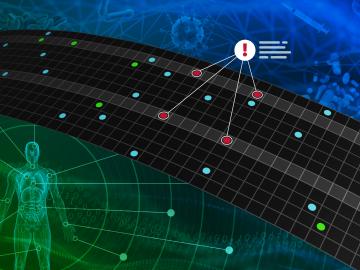
A team of researchers has developed a novel, machine learning–based technique to explore and identify relationships among medical concepts using electronic health record data across multiple healthcare providers.