Filter News
Area of Research
- (-) National Security (21)
- (-) Nuclear Science and Technology (1)
- (-) Supercomputing (40)
- Advanced Manufacturing (2)
- Biology and Environment (18)
- Clean Energy (38)
- Climate and Environmental Systems (1)
- Computational Engineering (1)
- Computer Science (9)
- Electricity and Smart Grid (1)
- Functional Materials for Energy (2)
- Fusion and Fission (9)
- Fusion Energy (5)
- Isotopes (2)
- Materials (32)
- Materials for Computing (4)
- Neutron Science (9)
- Quantum information Science (3)
News Topics
- (-) Big Data (9)
- (-) Computer Science (38)
- (-) Critical Materials (1)
- (-) Cybersecurity (8)
- (-) Energy Storage (4)
- (-) Fusion (1)
- (-) Grid (7)
- (-) Machine Learning (9)
- (-) Microscopy (2)
- 3-D Printing/Advanced Manufacturing (2)
- Advanced Reactors (4)
- Artificial Intelligence (16)
- Bioenergy (5)
- Biology (7)
- Biomedical (6)
- Biotechnology (1)
- Buildings (3)
- Chemical Sciences (2)
- Climate Change (7)
- Coronavirus (4)
- Decarbonization (2)
- Environment (6)
- Exascale Computing (8)
- Frontier (9)
- High-Performance Computing (9)
- Materials (8)
- Materials Science (6)
- Nanotechnology (4)
- National Security (13)
- Neutron Science (3)
- Nuclear Energy (11)
- Partnerships (1)
- Physics (3)
- Quantum Computing (7)
- Quantum Science (8)
- Security (6)
- Simulation (5)
- Space Exploration (5)
- Summit (16)
- Sustainable Energy (4)
- Transportation (1)
Media Contacts
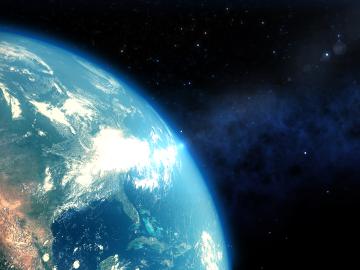
Tackling the climate crisis and achieving an equitable clean energy future are among the biggest challenges of our time.

Unequal access to modern infrastructure is a feature of growing cities, according to a study published this week in the Proceedings of the National Academy of Sciences

ORNL scientists had a problem mapping the genomes of bacteria to better understand the origins of their physical traits and improve their function for bioenergy production.
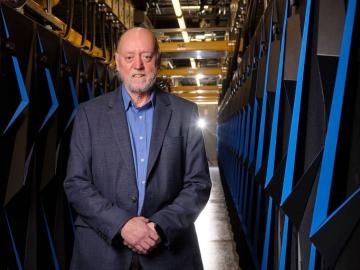
A force within the supercomputing community, Jack Dongarra developed software packages that became standard in the industry, allowing high-performance computers to become increasingly more powerful in recent decades.
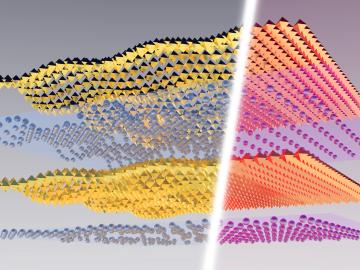
A study by researchers at the ORNL takes a fresh look at what could become the first step toward a new generation of solar batteries.

More than 50 current employees and recent retirees from ORNL received Department of Energy Secretary’s Honor Awards from Secretary Jennifer Granholm in January as part of project teams spanning the national laboratory system. The annual awards recognized 21 teams and three individuals for service and contributions to DOE’s mission and to the benefit of the nation.
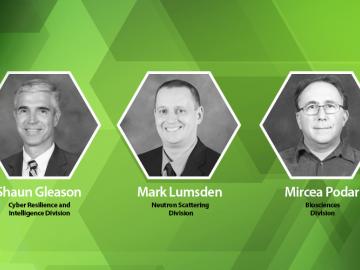
Three ORNL scientists have been elected fellows of the American Association for the Advancement of Science, or AAAS, the world’s largest general scientific society and publisher of the Science family of journals.

A new version of the Energy Exascale Earth System Model, or E3SM, is two times faster than an earlier version released in 2018.

A team of scientists led by the Department of Energy’s Oak Ridge National Laboratory and the Georgia Institute of Technology is using supercomputing and revolutionary deep learning tools to predict the structures and roles of thousands of proteins with unknown functions.
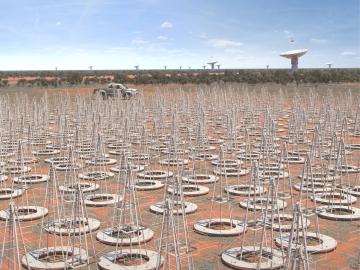
For nearly three decades, scientists and engineers across the globe have worked on the Square Kilometre Array (SKA), a project focused on designing and building the world’s largest radio telescope. Although the SKA will collect enormous amounts of precise astronomical data in record time, scientific breakthroughs will only be possible with systems able to efficiently process that data.