Filter News
Area of Research
News Topics
- (-) Energy Storage (1)
- (-) Materials Science (2)
- 3-D Printing/Advanced Manufacturing (1)
- Advanced Reactors (1)
- Artificial Intelligence (2)
- Chemical Sciences (1)
- Clean Water (2)
- Computer Science (3)
- Environment (4)
- High-Performance Computing (1)
- Isotopes (1)
- Materials (4)
- Microscopy (1)
- Nanotechnology (2)
- Neutron Science (1)
- Nuclear Energy (4)
- Physics (1)
- Sustainable Energy (1)
- Transportation (1)
Media Contacts

A study led by researchers at ORNL could help make materials design as customizable as point-and-click.
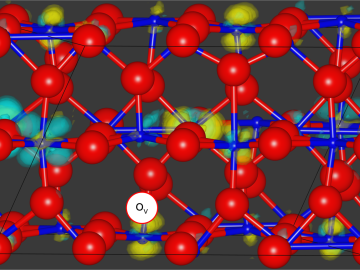
Neuromorphic devices — which emulate the decision-making processes of the human brain — show great promise for solving pressing scientific problems, but building physical systems to realize this potential presents researchers with a significant
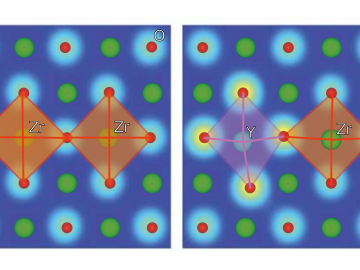
Ionic conduction involves the movement of ions from one location to another inside a material. The ions travel through point defects, which are irregularities in the otherwise consistent arrangement of atoms known as the crystal lattice. This sometimes sluggish process can limit the performance and efficiency of fuel cells, batteries, and other energy storage technologies.