Filter News
Area of Research
News Topics
- (-) Artificial Intelligence (1)
- (-) Big Data (1)
- (-) Chemical Sciences (2)
- (-) Frontier (1)
- (-) Polymers (1)
- Bioenergy (11)
- Biology (16)
- Biomedical (1)
- Biotechnology (2)
- Clean Water (1)
- Climate Change (13)
- Composites (1)
- Computer Science (2)
- Coronavirus (1)
- Decarbonization (7)
- Environment (17)
- Exascale Computing (1)
- High-Performance Computing (2)
- Hydropower (3)
- Machine Learning (2)
- Materials (1)
- Materials Science (1)
- Mercury (1)
- Microscopy (5)
- Nanotechnology (1)
- Net Zero (1)
- Simulation (1)
- Summit (2)
- Sustainable Energy (6)
Media Contacts
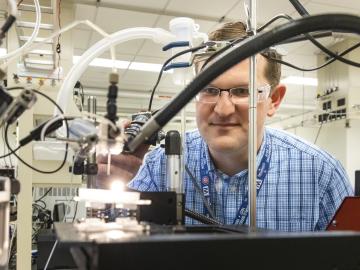
John “Jack” Cahill is out to illuminate previously unseen processes with new technology, advancing our understanding of how chemicals interact to influence complex systems whether it’s in the human body or in the world beneath our feet.
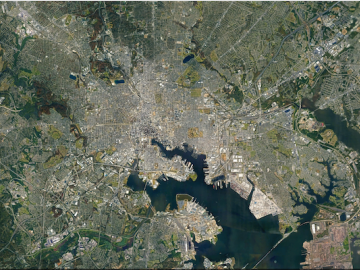
ORNL researchers are deploying their broad expertise in climate data and modeling to create science-based mitigation strategies for cities stressed by climate change as part of two U.S. Department of Energy Urban Integrated Field Laboratory projects.
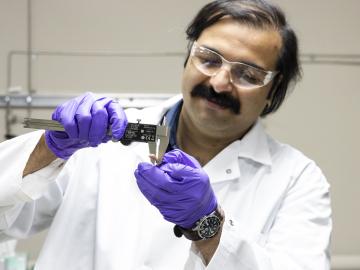
Chemical and environmental engineer Samarthya Bhagia is focused on achieving carbon neutrality and a circular economy by designing new plant-based materials for a range of applications from energy storage devices and sensors to environmentally friendly bioplastics.

The Atmospheric Radiation Measurement Data Center is shepherding changes to its operations to make the treasure trove of data more easily available accessible and useful to scientists studying Earth’s climate.

A team of scientists led by the Department of Energy’s Oak Ridge National Laboratory and the Georgia Institute of Technology is using supercomputing and revolutionary deep learning tools to predict the structures and roles of thousands of proteins with unknown functions.