Filter News
Area of Research
- (-) Supercomputing (19)
- Biology and Environment (23)
- Biology and Soft Matter (1)
- Clean Energy (14)
- Climate and Environmental Systems (1)
- Computer Science (1)
- Electricity and Smart Grid (1)
- Energy Frontier Research Centers (1)
- Functional Materials for Energy (1)
- Fusion and Fission (2)
- Isotopes (1)
- Materials (17)
- Materials for Computing (4)
- National Security (11)
- Neutron Science (5)
- Nuclear Science and Technology (1)
- Sensors and Controls (1)
News Topics
- (-) Artificial Intelligence (8)
- (-) Big Data (3)
- (-) Climate Change (4)
- (-) Frontier (7)
- (-) Nanotechnology (3)
- (-) Security (2)
- Bioenergy (2)
- Biology (5)
- Biomedical (4)
- Buildings (3)
- Chemical Sciences (2)
- Computer Science (15)
- Coronavirus (3)
- Critical Materials (2)
- Cybersecurity (1)
- Decarbonization (2)
- Energy Storage (3)
- Environment (3)
- Exascale Computing (6)
- Grid (2)
- High-Performance Computing (8)
- Machine Learning (5)
- Materials (8)
- Materials Science (5)
- Microscopy (2)
- National Security (3)
- Neutron Science (1)
- Partnerships (1)
- Physics (2)
- Polymers (1)
- Quantum Computing (7)
- Quantum Science (5)
- Simulation (5)
- Space Exploration (1)
- Summit (9)
- Sustainable Energy (2)
- Transportation (1)
Media Contacts

A study led by researchers at ORNL could help make materials design as customizable as point-and-click.
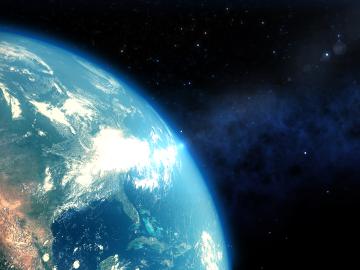
Tackling the climate crisis and achieving an equitable clean energy future are among the biggest challenges of our time.
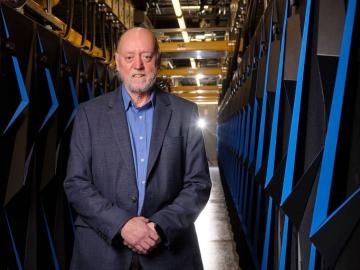
A force within the supercomputing community, Jack Dongarra developed software packages that became standard in the industry, allowing high-performance computers to become increasingly more powerful in recent decades.
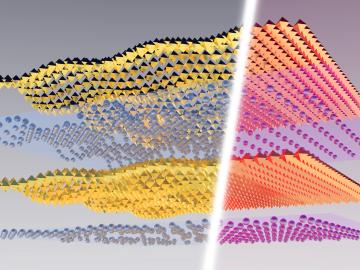
A study by researchers at the ORNL takes a fresh look at what could become the first step toward a new generation of solar batteries.

More than 50 current employees and recent retirees from ORNL received Department of Energy Secretary’s Honor Awards from Secretary Jennifer Granholm in January as part of project teams spanning the national laboratory system. The annual awards recognized 21 teams and three individuals for service and contributions to DOE’s mission and to the benefit of the nation.
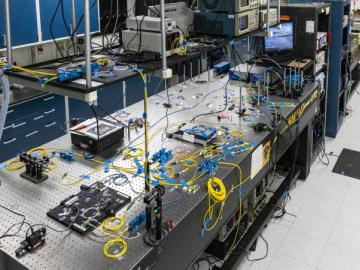
A rapidly emerging consensus in the scientific community predicts the future will be defined by humanity’s ability to exploit the laws of quantum mechanics.

A new version of the Energy Exascale Earth System Model, or E3SM, is two times faster than an earlier version released in 2018.

A team of scientists led by the Department of Energy’s Oak Ridge National Laboratory and the Georgia Institute of Technology is using supercomputing and revolutionary deep learning tools to predict the structures and roles of thousands of proteins with unknown functions.
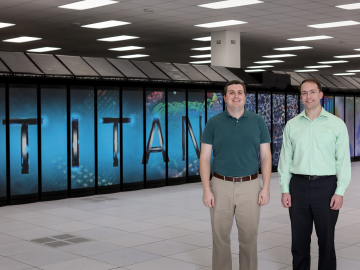
A team of researchers from the Department of Energy’s Oak Ridge National Laboratory has married artificial intelligence and high-performance computing to achieve a peak speed of 20 petaflops in the generation and training of deep learning networks on the