
Filter News
Area of Research
News Topics
- (-) Chemical Sciences (15)
- (-) Machine Learning (10)
- 3-D Printing/Advanced Manufacturing (12)
- Advanced Reactors (4)
- Artificial Intelligence (14)
- Big Data (9)
- Bioenergy (19)
- Biology (28)
- Biomedical (6)
- Biotechnology (3)
- Buildings (16)
- Clean Water (5)
- Climate Change (26)
- Composites (3)
- Computer Science (20)
- Coronavirus (9)
- Critical Materials (4)
- Cybersecurity (7)
- Decarbonization (21)
- Element Discovery (1)
- Energy Storage (25)
- Environment (36)
- Exascale Computing (8)
- Fossil Energy (1)
- Frontier (10)
- Fusion (7)
- Grid (13)
- High-Performance Computing (16)
- Hydropower (8)
- Irradiation (1)
- Isotopes (4)
- ITER (2)
- Materials (37)
- Materials Science (16)
- Mercury (1)
- Microscopy (13)
- Nanotechnology (9)
- National Security (17)
- Net Zero (2)
- Neutron Science (12)
- Nuclear Energy (10)
- Partnerships (8)
- Physics (10)
- Polymers (5)
- Quantum Computing (7)
- Quantum Science (9)
- Security (4)
- Simulation (6)
- Space Exploration (4)
- Summit (7)
- Sustainable Energy (25)
- Transformational Challenge Reactor (2)
- Transportation (10)
Media Contacts
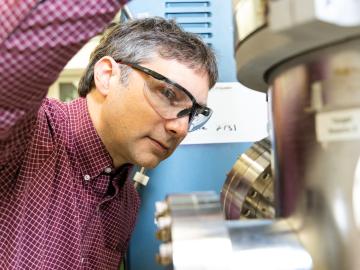
Several electrolyte and thin-film coating technologies, developed at Oak Ridge National Laboratory, have been licensed by BTRY, a battery technology company based in Virginia, to make batteries with increased energy density, at lower cost, and with an improved safety profile in crashes.

ORNL biogeochemist Elizabeth Herndon is working with colleagues to investigate a piece of the puzzle that has received little attention thus far: the role of manganese in the carbon cycle.

ORNL scientists had a problem mapping the genomes of bacteria to better understand the origins of their physical traits and improve their function for bioenergy production.
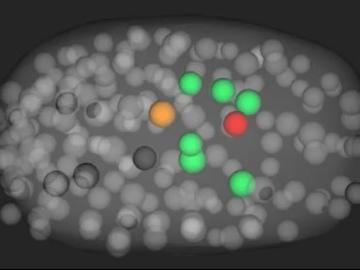
Scientists have developed a novel approach to computationally infer previously undetected behaviors within complex biological environments by analyzing live, time-lapsed images that show the positioning of embryonic cells in C. elegans, or roundworms. Their published methods could be used to reveal hidden biological activity.

A team of scientists led by the Department of Energy’s Oak Ridge National Laboratory and the Georgia Institute of Technology is using supercomputing and revolutionary deep learning tools to predict the structures and roles of thousands of proteins with unknown functions.