Filter News
Area of Research
- Advanced Manufacturing (3)
- Biological Systems (1)
- Biology and Environment (21)
- Clean Energy (58)
- Computational Biology (1)
- Electricity and Smart Grid (1)
- Fusion and Fission (20)
- Fusion Energy (4)
- Isotopes (5)
- Materials (23)
- Materials for Computing (2)
- National Security (14)
- Neutron Science (12)
- Nuclear Science and Technology (16)
- Supercomputing (30)
News Type
News Topics
- (-) 3-D Printing/Advanced Manufacturing (33)
- (-) Advanced Reactors (6)
- (-) Artificial Intelligence (38)
- (-) Biomedical (26)
- (-) Clean Water (14)
- (-) Grid (21)
- (-) Nuclear Energy (49)
- (-) Security (10)
- (-) Transportation (25)
- Big Data (21)
- Bioenergy (46)
- Biology (53)
- Biotechnology (9)
- Buildings (16)
- Chemical Sciences (19)
- Climate Change (44)
- Composites (5)
- Computer Science (76)
- Coronavirus (17)
- Critical Materials (1)
- Cybersecurity (14)
- Decarbonization (39)
- Emergency (2)
- Energy Storage (28)
- Environment (98)
- Exascale Computing (21)
- Fossil Energy (3)
- Frontier (19)
- Fusion (27)
- High-Performance Computing (38)
- Hydropower (5)
- Isotopes (23)
- ITER (2)
- Machine Learning (19)
- Materials (37)
- Materials Science (37)
- Mathematics (5)
- Mercury (7)
- Microelectronics (2)
- Microscopy (19)
- Molten Salt (1)
- Nanotechnology (16)
- National Security (30)
- Net Zero (6)
- Neutron Science (43)
- Partnerships (11)
- Physics (25)
- Polymers (7)
- Quantum Computing (15)
- Quantum Science (24)
- Renewable Energy (1)
- Simulation (25)
- Software (1)
- Space Exploration (11)
- Summit (29)
- Sustainable Energy (37)
- Transformational Challenge Reactor (3)
Media Contacts
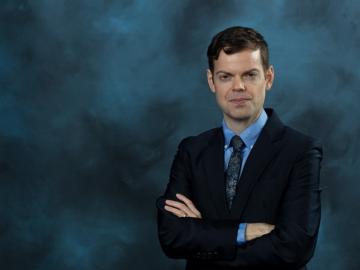
While government regulations are slowly coming, a group of cybersecurity professionals are sharing best practices to protect large language models powering these tools. Sean Oesch, a leader in emerging cyber technologies, recently contributed to the OWASP AI Security and Privacy Guide to inform global AI security standards and regulations.
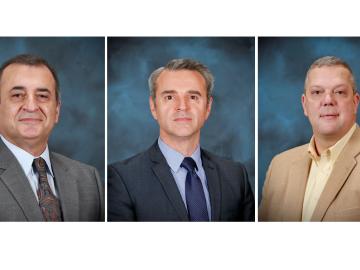
Three staff members in ORNL’s Fusion and Fission Energy and Science Directorate have moved into newly established roles facilitating communication and program management with sponsors of the directorate’s Nuclear Energy and Fuel Cycle Division.

Scientists at ORNL are looking for a happy medium to enable the grid of the future, filling a gap between high and low voltages for power electronics technology that underpins the modern U.S. electric grid.

Researchers at the Department of Energy’s Oak Ridge and Lawrence Berkeley National Laboratories are evolving graph neural networks to scale on the nation’s most powerful computational resources, a necessary step in tackling today’s data-centric

New computational framework speeds discovery of fungal metabolites, key to plant health and used in drug therapies and for other uses.

In summer 2023, ORNL's Prasanna Balaprakash was invited to speak at a roundtable discussion focused on the importance of academic artificial intelligence research and development hosted by the White House Office of Science and Technology Policy and the U.S. National Science Foundation.
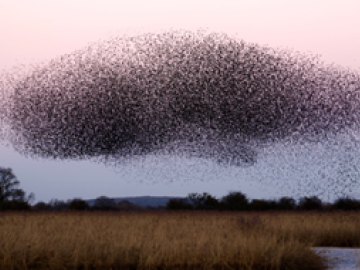
A team of researchers from the University of Southern California, the Renaissance Computing Institute at the University of North Carolina, and Oak Ridge, Lawrence Berkeley and Argonne National Laboratories have received a grant from the U.S. Department of Energy to develop the fundamentals of a computational platform that is fault tolerant, robust to various environmental conditions and adaptive to workloads and resource availability.
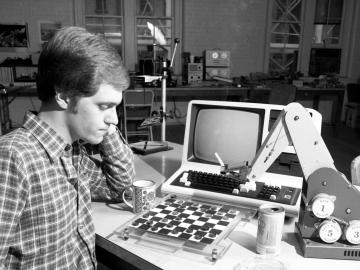
Despite its futuristic essence, artificial intelligence has a history that can be traced through several decades, and the ORNL has played a major role. From helping to drive fundamental and applied AI research from the field’s early days focused on expert systems, computer programs that rely on AI, to more recent developments in deep learning, a form of AI that enables machines to make evidence-based decisions, the lab’s AI research spans the spectrum.
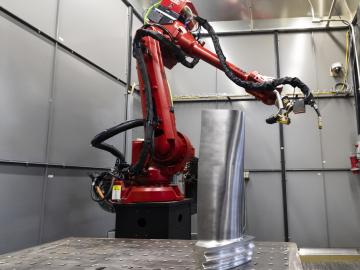
Researchers at ORNL became the first to 3D-print large rotating steam turbine blades for generating energy in power plants.
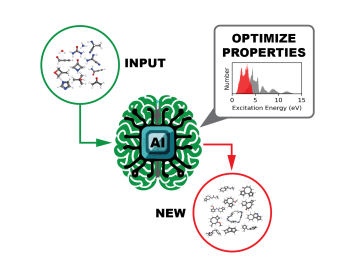
A team of computational scientists at ORNL has generated and released datasets of unprecedented scale that provide the ultraviolet visible spectral properties of over 10 million organic molecules.