
Filter News
Area of Research
- Biology and Environment (14)
- Clean Energy (35)
- Computer Science (1)
- Electricity and Smart Grid (1)
- Fusion and Fission (2)
- Isotopes (2)
- Materials (14)
- Materials for Computing (3)
- National Security (17)
- Neutron Science (7)
- Nuclear Science and Technology (1)
- Quantum information Science (2)
- Supercomputing (13)
News Type
News Topics
- (-) Cybersecurity (14)
- (-) Energy Storage (30)
- (-) Grid (26)
- (-) Machine Learning (23)
- (-) Microscopy (20)
- (-) Space Exploration (12)
- 3-D Printing/Advanced Manufacturing (43)
- Advanced Reactors (8)
- Artificial Intelligence (51)
- Big Data (30)
- Bioenergy (51)
- Biology (61)
- Biomedical (32)
- Biotechnology (12)
- Buildings (23)
- Chemical Sciences (28)
- Clean Water (15)
- Climate Change (52)
- Composites (8)
- Computer Science (89)
- Coronavirus (17)
- Critical Materials (5)
- Decarbonization (47)
- Education (2)
- Emergency (2)
- Environment (105)
- Exascale Computing (31)
- Fossil Energy (4)
- Frontier (27)
- Fusion (31)
- High-Performance Computing (49)
- Hydropower (5)
- Isotopes (31)
- ITER (2)
- Materials (46)
- Materials Science (49)
- Mathematics (7)
- Mercury (7)
- Microelectronics (3)
- Molten Salt (1)
- Nanotechnology (16)
- National Security (47)
- Net Zero (8)
- Neutron Science (54)
- Nuclear Energy (56)
- Partnerships (21)
- Physics (31)
- Polymers (8)
- Quantum Computing (23)
- Quantum Science (32)
- Renewable Energy (1)
- Security (12)
- Simulation (34)
- Software (1)
- Statistics (1)
- Summit (33)
- Sustainable Energy (48)
- Transformational Challenge Reactor (3)
- Transportation (27)
Media Contacts
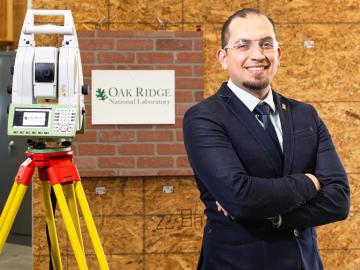
As a mechanical engineer in building envelope materials research at ORNL, Bryan Maldonado sees opportunities to apply his scientific expertise virtually everywhere he goes, from coast to coast. As an expert in understanding how complex systems operate, he’s using machine learning methods to control the process and ultimately optimize performance.
Researchers at ORNL recently demonstrated an automated drone-inspection technology at EPB of Chattanooga that will allow utilities to more quickly and easily check remote power lines for malfunctions, catching problems before outages occur.

At ORNL, a group of scientists used neutron scattering techniques to investigate a relatively new functional material called a Weyl semimetal. These Weyl fermions move very quickly in a material and can carry electrical charge at room temperature. Scientists think that Weyl semimetals, if used in future electronics, could allow electricity to flow more efficiently and enable more energy-efficient computers and other electronic devices.
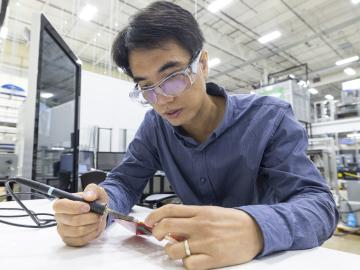
Researchers at the Department of Energy’s Oak Ridge National Laboratory and partner institutions have launched a project to develop an innovative suite of tools that will employ machine learning algorithms for more effective cybersecurity analysis of the U.S. power grid.

Power companies and electric grid developers turn to simulation tools as they attempt to understand how modern equipment will be affected by rapidly unfolding events in a complex grid.

ORNL's Guang Yang and Andrew Westover have been selected to join the first cohort of DOE’s Advanced Research Projects Agency-Energy Inspiring Generations of New Innovators to Impact Technologies in Energy 2024 program. The program supports early career scientists and engineers in their work to convert disruptive ideas into impactful energy technologies.
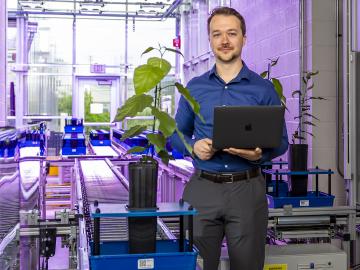
John Lagergren, a staff scientist in Oak Ridge National Laboratory’s Plant Systems Biology group, is using his expertise in applied math and machine learning to develop neural networks to quickly analyze the vast amounts of data on plant traits amassed at ORNL’s Advanced Plant Phenotyping Laboratory.
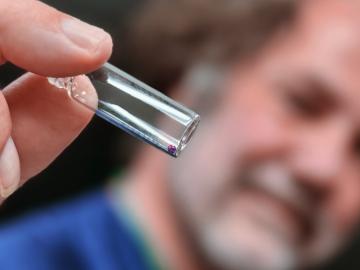
Researchers set a new benchmark for future experiments making materials in space rather than for space. They discovered that many kinds of glass have similar atomic structure and arrangements and can successfully be made in space. Scientists from nine institutions in government, academia and industry participated in this 5-year study.
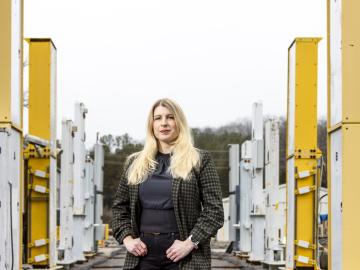
Researchers tackling national security challenges at ORNL are upholding an 80-year legacy of leadership in all things nuclear. Today, they’re developing the next generation of technologies that will help reduce global nuclear risk and enable safe, secure, peaceful use of nuclear materials, worldwide.
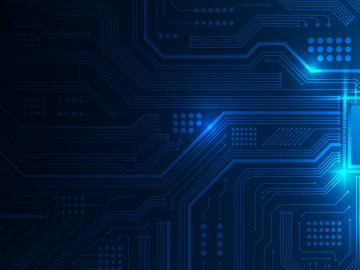
A team led by researchers at ORNL explored training strategies for one of the largest artificial intelligence models to date with help from the world’s fastest supercomputer. The findings could help guide training for a new generation of AI models for scientific research.