Filter Results
Related Organization
- Biological and Environmental Systems Science Directorate (23)
- Computing and Computational Sciences Directorate (35)
- Energy Science and Technology Directorate (217)
- Fusion and Fission Energy and Science Directorate (21)
- Information Technology Services Directorate (2)
- Isotope Science and Enrichment Directorate (6)
- National Security Sciences Directorate (17)
- Neutron Sciences Directorate (11)
- Physical Sciences Directorate (128)
- User Facilities
(27)
Researcher
- Srikanth Yoginath
- Costas Tsouris
- Gs Jung
- Gyoung Gug Jang
- James J Nutaro
- Pratishtha Shukla
- Radu Custelcean
- Sudip Seal
- Alexander I Wiechert
- Ali Passian
- Bogdan Dryzhakov
- Christopher Rouleau
- Debangshu Mukherjee
- Harper Jordan
- Ilia N Ivanov
- Ivan Vlassiouk
- Joel Asiamah
- Joel Dawson
- Jong K Keum
- Kyle Kelley
- Md Inzamam Ul Haque
- Mina Yoon
- Nance Ericson
- Olga S Ovchinnikova
- Pablo Moriano Salazar
- Steven Randolph
- Varisara Tansakul
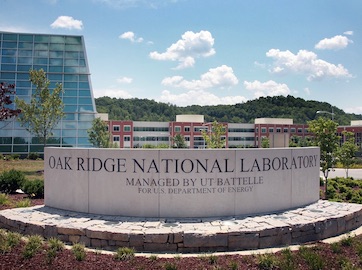
Among the methods for point source carbon capture, the absorption of CO2 using aqueous amines (namely MEA) from the post-combustion gas stream is currently considered the most promising.

High coercive fields prevalent in wurtzite ferroelectrics present a significant challenge, as they hinder efficient polarization switching, which is essential for microelectronic applications.

Digital twins (DTs) have emerged as essential tools for monitoring, predicting, and optimizing physical systems by using real-time data.

Simulation cloning is a technique in which dynamically cloned simulations’ state spaces differ from their parent simulation due to intervening events.
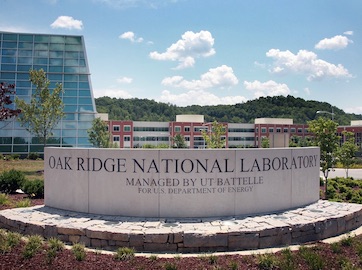
This technology is a laser-based heating unit that offers rapid heating profiles on a research scale with minimal incidental heating of materials processing environments.
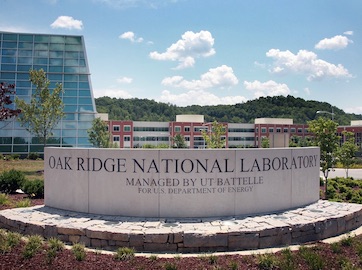
A novel molecular sorbent system for low energy CO2 regeneration is developed by employing CO2-responsive molecules and salt in aqueous media where a precipitating CO2--salt fractal network is formed, resulting in solid-phase formation and sedimentation.

This innovative approach combines optical and spectral imaging data via machine learning to accurately predict cancer labels directly from tissue images.