Filter Results
Related Organization
- Biological and Environmental Systems Science Directorate (26)
- Computing and Computational Sciences Directorate (38)
- Energy Science and Technology Directorate (223)
- Fusion and Fission Energy and Science Directorate
(24)
- Information Technology Services Directorate (3)
- Isotope Science and Enrichment Directorate (7)
- National Security Sciences Directorate (20)
- Neutron Sciences Directorate (11)
- Physical Sciences Directorate (135)
- User Facilities (27)
Researcher
- Eddie Lopez Honorato
- Ryan Heldt
- Tyler Gerczak
- Alexander I Wiechert
- Alexandre Sorokine
- Christopher Hobbs
- Clinton Stipek
- Costas Tsouris
- Daniel Adams
- Debangshu Mukherjee
- Gs Jung
- Gyoung Gug Jang
- Jessica Moehl
- Matt Kurley III
- Md Inzamam Ul Haque
- Olga S Ovchinnikova
- Philipe Ambrozio Dias
- Radu Custelcean
- Rodney D Hunt
- Taylor Hauser
- Viswadeep Lebakula
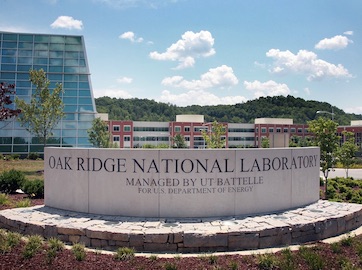
Understanding building height is imperative to the overall study of energy efficiency, population distribution, urban morphologies, emergency response, among others. Currently, existing approaches for modelling building height at scale are hindered by two pervasive issues.
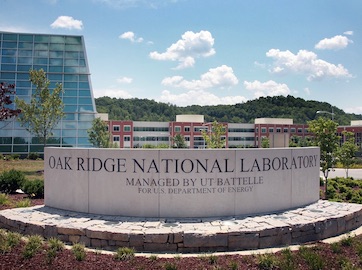
Among the methods for point source carbon capture, the absorption of CO2 using aqueous amines (namely MEA) from the post-combustion gas stream is currently considered the most promising.
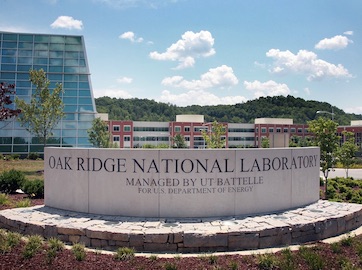
Sintering additives to improve densification and microstructure control of UN provides a facile approach to producing high quality nuclear fuels.
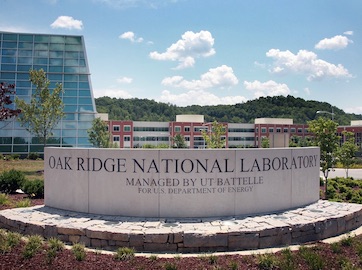
In order to avoid the limitations and costs due to the use of monolithic components for chemical vapor deposition, we developed a modular system in which the reaction chamber can be composed of a top and bottom cone, nozzle, and in-situ reaction chambers.
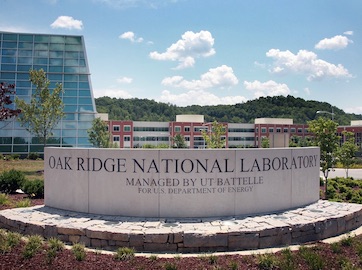
The use of Fluidized Bed Chemical Vapor Deposition to coat particles or fibers is inherently slow and capital intensive, as it requires constant modifications to the equipment to account for changes in the characteristics of the substrates to be coated.

This innovative approach combines optical and spectral imaging data via machine learning to accurately predict cancer labels directly from tissue images.