Filter Results
Related Organization
- Biological and Environmental Systems Science Directorate (26)
- Computing and Computational Sciences Directorate (38)
- Energy Science and Technology Directorate (223)
- Fusion and Fission Energy and Science Directorate (24)
- Information Technology Services Directorate (3)
- Isotope Science and Enrichment Directorate (7)
- National Security Sciences Directorate (20)
- Neutron Sciences Directorate (11)
- Physical Sciences Directorate (135)
- User Facilities (27)
Researcher
- Peeyush Nandwana
- Blane Fillingim
- Brian Post
- Lauren Heinrich
- Sudarsanam Babu
- Thomas Feldhausen
- Yousub Lee
- Alexander I Wiechert
- Alexandre Sorokine
- Bryan Lim
- Clinton Stipek
- Costas Tsouris
- Daniel Adams
- Debangshu Mukherjee
- Gs Jung
- Gyoung Gug Jang
- Jessica Moehl
- Md Inzamam Ul Haque
- Olga S Ovchinnikova
- Philipe Ambrozio Dias
- Radu Custelcean
- Ramanan Sankaran
- Rangasayee Kannan
- Taylor Hauser
- Tomas Grejtak
- Vimal Ramanuj
- Viswadeep Lebakula
- Wenjun Ge
- Yiyu Wang
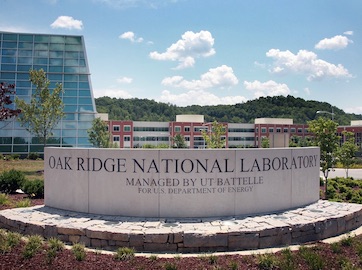
Understanding building height is imperative to the overall study of energy efficiency, population distribution, urban morphologies, emergency response, among others. Currently, existing approaches for modelling building height at scale are hindered by two pervasive issues.
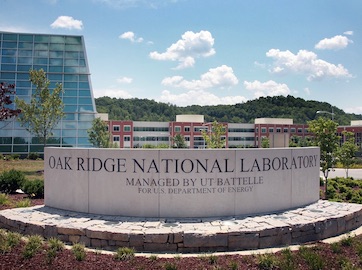
Among the methods for point source carbon capture, the absorption of CO2 using aqueous amines (namely MEA) from the post-combustion gas stream is currently considered the most promising.
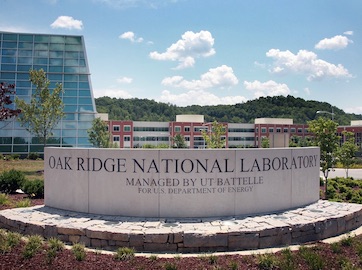
A new nanostructured bainitic steel with accelerated kinetics for bainite formation at 200 C was designed using a coupled CALPHAD, machine learning, and data mining approach.
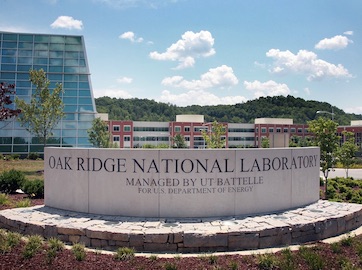
This work seeks to alter the interface condition through thermal history modification, deposition energy density, and interface surface preparation to prevent interface cracking.
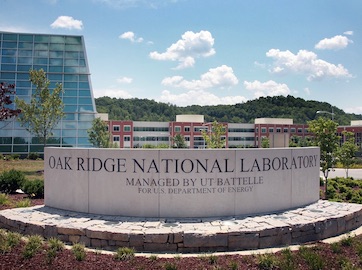
Additive manufacturing (AM) enables the incremental buildup of monolithic components with a variety of materials, and material deposition locations.
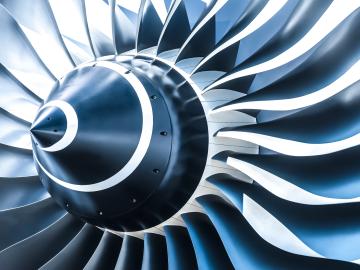
Ceramic matrix composites are used in several industries, such as aerospace, for lightweight, high quality and high strength materials. But producing them is time consuming and often low quality.

This innovative approach combines optical and spectral imaging data via machine learning to accurately predict cancer labels directly from tissue images.