Filter Results
Related Organization
- Biological and Environmental Systems Science Directorate (26)
- Computing and Computational Sciences Directorate (38)
- Energy Science and Technology Directorate
(223)
- Fusion and Fission Energy and Science Directorate (24)
- Information Technology Services Directorate (3)
- Isotope Science and Enrichment Directorate (7)
- National Security Sciences Directorate (20)
- Neutron Sciences Directorate (11)
- Physical Sciences Directorate (135)
- User Facilities (27)
Researcher
- Venkatakrishnan Singanallur Vaidyanathan
- Amir K Ziabari
- Chad Steed
- Junghoon Chae
- Mingyan Li
- Philip Bingham
- Ryan Dehoff
- Sam Hollifield
- Travis Humble
- Vincent Paquit
- Brian Weber
- Diana E Hun
- Femi Omitaomu
- Gina Accawi
- Gurneesh Jatana
- Haowen Xu
- Isaac Sikkema
- Joseph Olatt
- Kevin Spakes
- Kunal Mondal
- Lilian V Swann
- Luke Koch
- Mahim Mathur
- Mark M Root
- Mary A Adkisson
- Michael Kirka
- Obaid Rahman
- Oscar Martinez
- Philip Boudreaux
- Samudra Dasgupta
- T Oesch
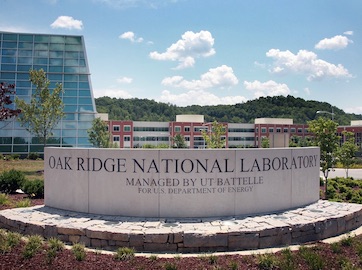
ORNL researchers have developed a deep learning-based approach to rapidly perform high-quality reconstructions from sparse X-ray computed tomography measurements.
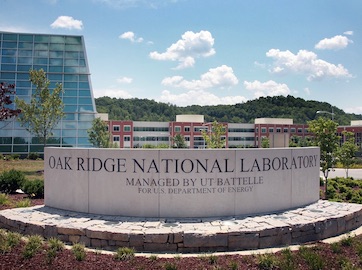
We have been working to adapt background oriented schlieren (BOS) imaging to directly visualize building leakage, which is fast and easy.
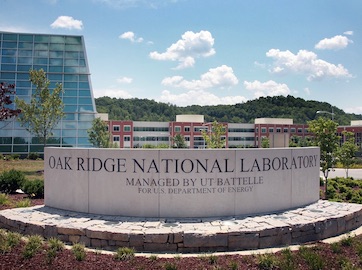
The QVis Quantum Device Circuit Optimization Module gives users the ability to map a circuit to a specific quantum devices based on the device specifications.
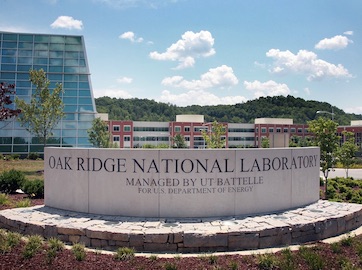
QVis is a visual analytics tool that helps uncover temporal and multivariate variations in noise properties of quantum devices.
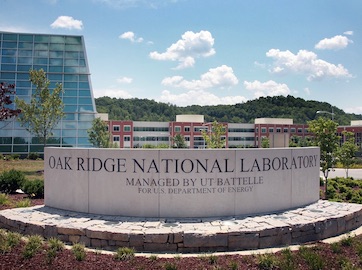
We will develop an AI-powered autonomous software development pipeline to help urban scientists develop advanced research software (e.g., digital twins and cyberinfrastructure) to support smart city research and management without the need to write codes or know software engin

Real-time tracking and monitoring of radioactive/nuclear materials during transportation is a critical need to ensure safety and security. Current technologies rely on simple tagging, using sensors attached to transport containers, but they have limitations.

Simurgh revolutionizes industrial CT imaging with AI, enhancing speed and accuracy in nondestructive testing for complex parts, reducing costs.