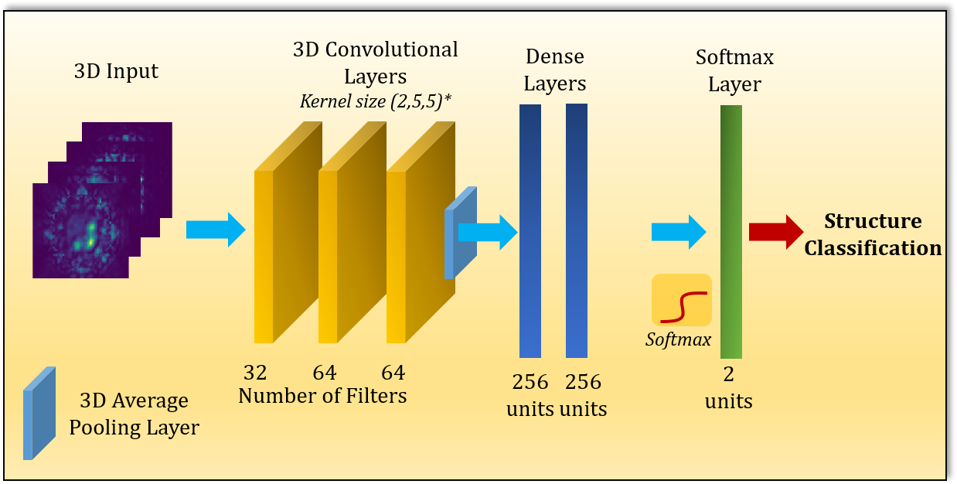
The interface at which materials meet can greatly impact performance. ORNL researchers married simulation and deep neural networks to distinguish structural features in the interface of advanced materials with potential to improve high-speed electronics.
An ORNL research team used deep neural networks to distinguish structural features of simulated Lanthanum Aluminate/Strontium Titanate (LaAlO3/SrTiO3) interfaces, tackling a long-standing and unsolved inverse problem.
Specifically, the team used ORNL’s Titan supercomputer to perform hundreds of electron diffraction simulations of various structures that could be feasibly encountered within electron microscopy experiments of the LaAlO3-SrTiO3 interface. They then leveraged the GPU acceleration provided by ORNL’s Summit system, currently the world’s most powerful, to train deep convolutional neural networks on the simulated data, allowing analysis of defining structural features in the technologically relevant oxide heterostructure. The method is general and can be readily applicable to structural determination problems across many distinct imaging modalities, and will be readily applied to data emanating from ORNL’s imaging user facilities such as the Center for Nanophase Materials Sciences, where this work was carried out.
Research team: Mark Oxley, Rama Vasudevan, Stephen Jesse, Andrew Lupini, Nikolay Borodinov, Junqi Yin, Sergei Kalinin
Funding: This research was supported by the Laboratory Directed Research and Development Program of ORNL, managed by UT-Battelle, LLC, for the U.S. DOE. This research was conducted at the Center for Nanophase Materials Sciences, which is a DOE office of Science User Facility. This research used resources of the Oak Ridge Leadership Computing Facility, which is a DOE Office of Science User Facility supported under Contract DE-AC05-00OR22725.
Publication: Nikolay Borodinov, Sabine Neumayer, Sergei V. Kalinin, Olga S. Ovchinnikova, Rama K. Vasudevan & Stephen Jesse. “Deep neural networks for understanding noisy data applied to physical property extraction in scanning probe microscopy.” npj Computational Materials volume 5, Article number: 25 (2019).
Contact: Rama Vasudevan, vasudevanrk@ornl.gov