Filter Results
Related Organization
- Biological and Environmental Systems Science Directorate (23)
- Computing and Computational Sciences Directorate (35)
- Energy Science and Technology Directorate
(217)
- Fusion and Fission Energy and Science Directorate (21)
- Information Technology Services Directorate (2)
- Isotope Science and Enrichment Directorate (6)
- National Security Sciences Directorate (17)
- Neutron Sciences Directorate (11)
- Physical Sciences Directorate (128)
- User Facilities (27)
Researcher
- Singanallur Venkatakrishnan
- Amir K Ziabari
- Diana E Hun
- Philip Bingham
- Ryan Dehoff
- Vincent Paquit
- Alex Roschli
- Easwaran Krishnan
- Erin Webb
- Evin Carter
- Gina Accawi
- Gurneesh Jatana
- James Manley
- Jamieson Brechtl
- Jeremy Malmstead
- Joe Rendall
- Karen Cortes Guzman
- Kashif Nawaz
- Kitty K Mccracken
- Kuma Sumathipala
- Mark M Root
- Mengjia Tang
- Michael Kirka
- Muneeshwaran Murugan
- Obaid Rahman
- Oluwafemi Oyedeji
- Philip Boudreaux
- Soydan Ozcan
- Tomonori Saito
- Tyler Smith
- Xianhui Zhao
- Zoriana Demchuk
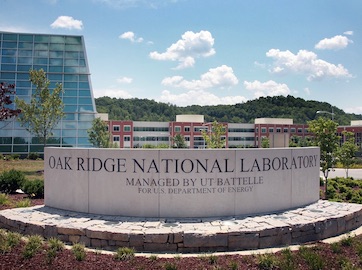
ORNL researchers have developed a deep learning-based approach to rapidly perform high-quality reconstructions from sparse X-ray computed tomography measurements.
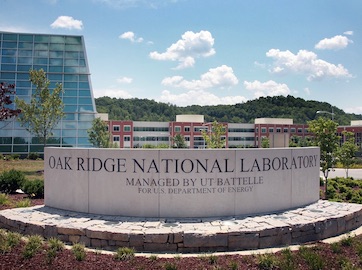
We have been working to adapt background oriented schlieren (BOS) imaging to directly visualize building leakage, which is fast and easy.
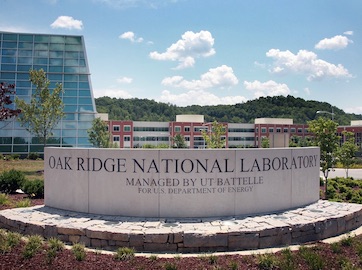
Estimates based on the U.S. Department of Energy (DOE) test procedure for water heaters indicate that the equivalent of 350 billion kWh worth of hot water is discarded annually through drains, and a large portion of this energy is, in fact, recoverable.
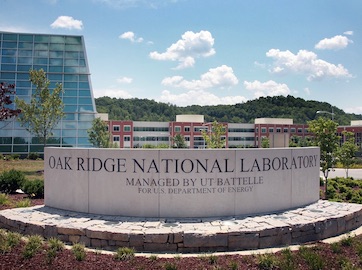
The use of biomass fiber reinforcement for polymer composite applications, like those in buildings or automotive, has expanded rapidly due to the low cost, high stiffness, and inherent renewability of these materials. Biomass are commonly disposed of as waste.
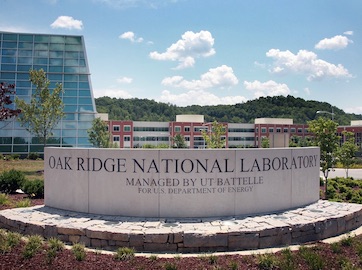
The incorporation of low embodied carbon building materials in the enclosure is increasing the fuel load for fire, increasing the demand for fire/flame retardants.

Simurgh revolutionizes industrial CT imaging with AI, enhancing speed and accuracy in nondestructive testing for complex parts, reducing costs.