Filter Results
Related Organization
- Biological and Environmental Systems Science Directorate (23)
- Computing and Computational Sciences Directorate (35)
- Energy Science and Technology Directorate (217)
- Fusion and Fission Energy and Science Directorate (21)
- Information Technology Services Directorate (2)
- Isotope Science and Enrichment Directorate (6)
- National Security Sciences Directorate (17)
- Neutron Sciences Directorate (11)
- Physical Sciences Directorate (128)
- User Facilities (27)
Researcher
- Ryan Dehoff
- Vincent Paquit
- Singanallur Venkatakrishnan
- William Carter
- Alex Roschli
- Amir K Ziabari
- Andrzej Nycz
- Brian Post
- Chris Masuo
- Diana E Hun
- Luke Meyer
- Peter Wang
- Philip Bingham
- Philip Boudreaux
- Stephen M Killough
- Adam Stevens
- Akash Jag Prasad
- Alex Walters
- Amy Elliott
- Bryan Maldonado Puente
- Calen Kimmell
- Cameron Adkins
- Canhai Lai
- Chris Tyler
- Clay Leach
- Corey Cooke
- Costas Tsouris
- Erin Webb
- Evin Carter
- Gina Accawi
- Gurneesh Jatana
- Isha Bhandari
- James Haley
- James Parks II
- Jaydeep Karandikar
- Jeremy Malmstead
- Joshua Vaughan
- Kitty K Mccracken
- Liam White
- Mark M Root
- Michael Borish
- Michael Kirka
- Nolan Hayes
- Obaid Rahman
- Oluwafemi Oyedeji
- Rangasayee Kannan
- Roger G Miller
- Ryan Kerekes
- Sally Ghanem
- Sarah Graham
- Soydan Ozcan
- Sudarsanam Babu
- Tyler Smith
- Vladimir Orlyanchik
- William Peter
- Xianhui Zhao
- Yukinori Yamamoto
- Zackary Snow
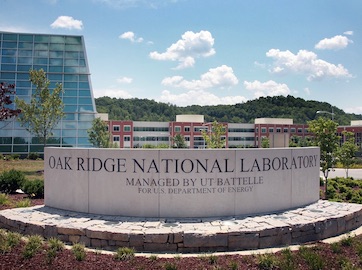
ORNL researchers have developed a deep learning-based approach to rapidly perform high-quality reconstructions from sparse X-ray computed tomography measurements.
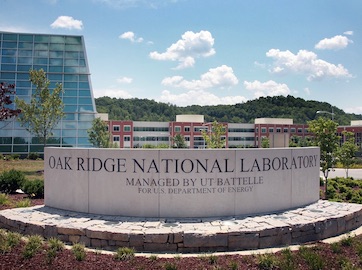
System and method for part porosity monitoring of additively manufactured components using machining
In additive manufacturing, choice of process parameters for a given material and geometry can result in porosities in the build volume, which can result in scrap.
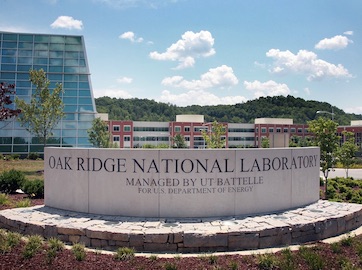
We have been working to adapt background oriented schlieren (BOS) imaging to directly visualize building leakage, which is fast and easy.
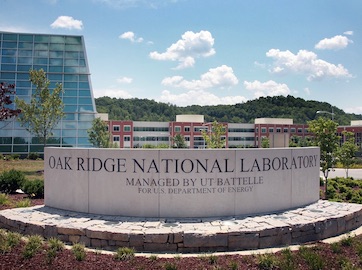
The use of biomass fiber reinforcement for polymer composite applications, like those in buildings or automotive, has expanded rapidly due to the low cost, high stiffness, and inherent renewability of these materials. Biomass are commonly disposed of as waste.
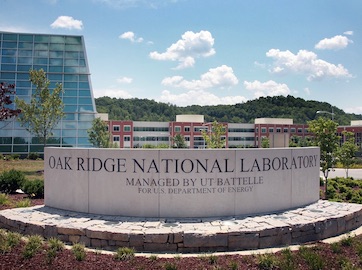
Sensing of additive manufacturing processes promises to facilitate detailed quality inspection at scales that have seldom been seen in traditional manufacturing processes.
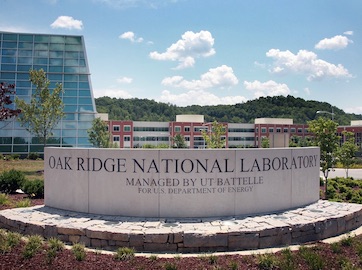
This invention utilizes new techniques in machine learning to accelerate the training of ML-based communication receivers.