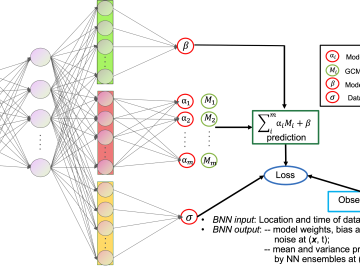
Multimodel ensembling improves predictions and considers model uncertainties. In this study, we present a Bayesian Neural Network (BNN) ensemble approach for large-scale precipitation predictions based on a set of climate models.
Multimodel ensembling improves predictions and considers model uncertainties. In this study, we present a Bayesian Neural Network (BNN) ensemble approach for large-scale precipitation predictions based on a set of climate models.
Automated experiments in Scanning Transmission Electron Microscopy (STEM) are implemented for rapid discovery of local structures, symmetry-breaking distortions, and internal electric and magnetic fields in complex materials.
Using first-principles calculations and group-theory-based models, we study the stabilization of ferrielectricity (FiE) in CuInP2Se6.
A multi-institutional team of ORNL has utilized the latest computational algorithms and parallelization techniques to enable faster than real-time simulations and applied it to the power system network whose time-domain model represents very large and h
Researchers from ORNL, Stanford University, and Purdue University developed and demonstrated a novel, fully functional quantum local area network (QLAN).
Researchers developed an automated scanning probe microscopy (SPM) platform to rapidly find regions of interest.
Quantum Monte Carlo (QMC) methods are used to find the structure and electronic band gap of 2D GeSe, determining that the gap and its nature are highly tunable by strain.
ORNL researchers developed a stochastic approximate gradient ascent method to reduce posterior uncertainty in Bayesian experimental design involving implicit models.
Dendritic solidification and microstructure evolution play a vital role in determining the material properties. Capturing the morphology of the solidification front becomes critical in predicting the final dendritic structure.