Brief: Graph neural networks and several optimizations are applied to predicting the CO2 adsorption of crystalline Meta-Organic Framework (MOF).
Accomplishment: We have developed a graph-based convolutional neural network approach for predicting and ranking gas adsorption properties of crystalline MOF adsorbents for application in post-combustion capture of CO2. Our model is based solely on standard structural input files containing atomistic descriptions of the adsorbent material candidates and can replace physics-based simulations and compute material properties in a fraction of the time and with a fraction of computational resources.
Description: We build our graph neural networks with reference to two existing architectures: Crystal Graph Convolutional Neural Network (CGCNN) and geometric Crystal Graph Neural Network (geoCGNN), with several enhancements that are critical to achieving state-of-the-art performance. The first optimization is that we incorporate a form of convolution for not only node features but also edge features. The second optimization is to introduce attention mechanism that automatically weighs the contribution from the incident edges and neighbors to a node’s feature. Multiheaded attention is also used in our Graph Neural Network (GNN). In addition to structural features, we also incorporate geometric features and partial charges into our GNN, which we call Enhanced Crystal Graph Convolutional Neural Network (ECGNN). In addition to these optimizations, we also explore recent techniques that make GNNs deeper without suffering from the over-smoothing deficiency of earlier GNN implementations. Interestingly, instead of performance improvement, we observe performance degradation. Several factors may contribute to this behavior. One of them could be related to the highly regular structure of MOFs. This suggests the need of specialized GNN development for scientific domains such as materials research.
We evaluate the performance of our various optimizations using standard MOF datasets such as CoRE-19. These datasets are curated for CO2 adsorption regression at two pressure points: 16 bar and .16 bar. The results are shown in the figure below for training and validation accuracies. It is clear that each optimization is effective, and combined together, they significantly improve performance.
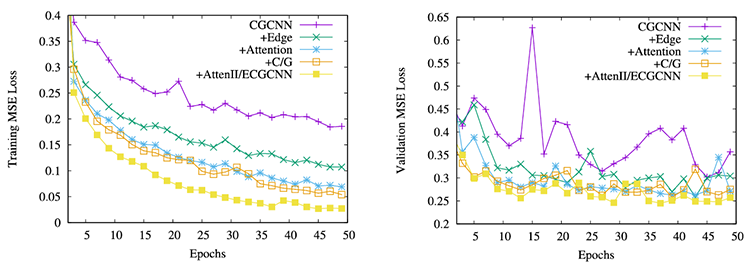
Acknowledgement: This research is funded by the AI Initiative, as part of the Laboratory Directed Research and Development Program of Oak Ridge National Laboratory, managed by UT-Battelle, LLC, for the U.S. Department of Energy (DOE).
Contact: Guojing Cong (congg@ornl.gov)
Team: Guojing Cong, Anshul Gupta, Rodrigo Neumann, Breanndan O Conchuir, with consultation from Matthew Ryder, and Shannon Mahurin
Publication: Prediction of CO2 Adsorption in Nano-Pores with Graph Neural Networks, the 7th International Workshop on Deep Learning on Graphs: Methods and Applications (DLG22) , part of the 36th AAAI Conference on Artificial Intelligence, A Virtual Conference, Feb 22 – March 1, 2022.