Robert Hettich: Decoding biological complexity with next-gen mass spectrometry
Filter News
Area of Research
News Type
News Topics
- (-) Grid (2)
- Artificial Intelligence (5)
- Big Data (4)
- Biology (2)
- Biotechnology (1)
- Buildings (1)
- Clean Water (1)
- Computer Science (4)
- Cybersecurity (1)
- Emergency (1)
- Environment (6)
- Exascale Computing (1)
- Frontier (2)
- High-Performance Computing (4)
- Hydropower (1)
- Machine Learning (4)
- Microscopy (1)
- National Security (4)
- Neutron Science (1)
- Nuclear Energy (1)
- Physics (2)
- Security (1)
- Simulation (4)
- Summit (1)
- Transportation (2)
Media Contacts
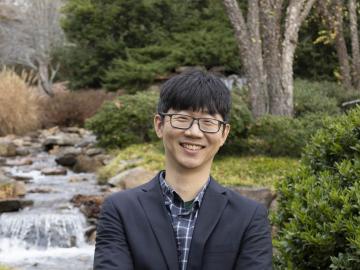
Lee's paper at the August conference in Bellevue, Washington, combined weather and power outage data for three states – Texas, Michigan and Hawaii – and used a machine learning model to predict how extreme weather such as thunderstorms, floods and tornadoes would affect local power grids and to estimate the risk for outages. The paper relied on data from the National Weather Service and the U.S. Department of Energy’s Environment for Analysis of Geo-Located Energy Information, or EAGLE-I, database.

Inspired by one of the mysteries of human perception, an ORNL researcher invented a new way to hide sensitive electric grid information from cyberattack: within a constantly changing color palette.