The 2021 Smoky Mountain Data Challenge included 55 teams, which were narrowed to 17 finalists, five award winners, four runners up and one honorable mention. Credit: ORNL, U.S. Dept. of Energy
This year’s virtual Smoky Mountains Computational Sciences and Engineering Conference, or SMC2021, an annual event hosted by the U.S. Department of Energy’s Oak Ridge National Laboratory, featured the fifth installment of the Data Challenge. Fifty-five teams — a record number for the data analysis competition — studied scientific data sets drawn from real-world research efforts by ORNL and industry data sponsors associated with eight brand-new challenges.
Teams of up to six members, which included student scientists, faculty and industry professionals, tackled predetermined tasks and answered increasingly difficult questions related to each challenge. Through this process, participants identified either partial solutions that demonstrated a better understanding of a topic or full solutions that provided the SMC program committee and the broader research community with new insights.
Six traditional data analytics challenges covered materials sciences, COVID-19 literature, supercomputer scheduling, sustainable cities, microscopy, and autonomous vehicles, a challenge that was sponsored by General Motors. The other two focused on neutron radiography and nanoscience in the context of edge computing, a field in which researchers analyze data generated by their instruments on the spot rather than relying on far-off data centers.
“The solutions that participants offered were innovative and exciting,” said Data Challenge Chair Pravallika Devineni, a research scientist at ORNL. “For example, a few teams used existing artificial intelligence solutions to improve the quality of imagery captured by autonomous vehicles under non-ideal weather conditions.”
From the 22 teams that submitted solutions to these problems, a panel of experts selected 17 finalists, who presented posters and three-minute lightning talks explaining their solutions during the conference. The top seven teams were invited to write papers describing their methods and findings for submission to SMC’s Springer conference proceedings following a peer-review process.
After viewing the lightning talks and participating in two-minute Q&A sessions, data sponsors and audience members filled out an online poll to determine the winners for awards in three categories: “Best Lightning Talk,” “Best Solution” and “People’s Choice.” The first two categories were subdivided into novice and advanced divisions and judged separately.
Winning teams, runners up and honorable mentions, which included individuals from high schools, universities, companies and national laboratories, received certificates commemorating their achievement.
Ridhima Singh of Farragut High School and Melissa Dumas and Nagendra Singh of ORNL received the “Best Lightning Talk” award in the novice category for “Exploring the Relationship between Demographic Indicators and the Built Environment of a City.” The runners up were Remington Kim of Bergen County Academies and Yue Ning of the Stevens Institute of Technology for “Recurrent Multi-task Graph Convolutional Networks for COVID-19 Knowledge Graph Link Prediction.” The awards were reversed for “Best Solution,” with Kim’s team earning the top prize and Singh’s team earning the runners up distinction.
In the advanced division of the “Best Lightning Talk” award, Lucas McCabe of George Washington University won for “Geodesic and Neural Features for Link Prediction in the COVID-19 Biomedical Knowledge Graph.” With “Finding Novel Links in the COVID-19 Knowledge Graph through Node Embedding Techniques,” Ankit Patel of Blue Wave AI Labs, Saeel Shrivallabh Pai and Manohar Bongarala of Purdue University, Haresh Rengaraj of New York University and Samyak Rajanala of Stanford University were runners up.
Patel’s team won the advanced version of the “Best Solution” award, and the runners up were Michael Lindstrom, William Swartworth and Deanna Needell of the University of California, Los Angeles for “Reconstructing Piezoelectric Responses over a Lattice: Adaptive Sampling of Low Dimensional Time Series Representations Based on Relative Isolation and Gradient Size.” ORNL’s Chen Zhang and Zachary Morgan received an honorable mention for “Advanced Image Reconstruction for MCP Detector in Event Mode.”
Finally, the “People’s Choice” winner was “Analyzing the Architectural, Geographic, and Demographic Distributions in Las Vegas, Nevada” by a team from the University of Tennessee at Chattanooga — Nyssa Hunt, Jin Cho, Jeremy Roland, Mina Sartipi and Mehdi Khaleghian.
UT-Battelle manages ORNL for DOE’s Office of Science, the single largest supporter of basic research in the physical sciences in the United States. DOE’s Office of Science is working to address some of the most pressing challenges of our time. For more information, visit https://energy.gov/science.— Elizabeth Rosenthal
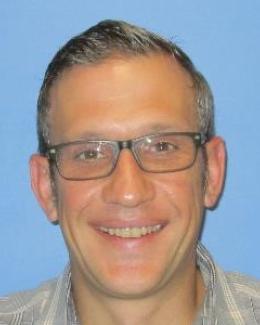