Filter News
Area of Research
News Topics
- (-) Emergency (4)
- (-) Software (1)
- 3-D Printing/Advanced Manufacturing (144)
- Advanced Reactors (40)
- Artificial Intelligence (125)
- Big Data (77)
- Bioenergy (110)
- Biology (126)
- Biomedical (73)
- Biotechnology (37)
- Buildings (73)
- Chemical Sciences (84)
- Clean Water (32)
- Composites (34)
- Computer Science (223)
- Coronavirus (48)
- Critical Materials (29)
- Cybersecurity (35)
- Education (5)
- Element Discovery (1)
- Energy Storage (114)
- Environment (217)
- Exascale Computing (64)
- Fossil Energy (8)
- Frontier (62)
- Fusion (65)
- Grid (74)
- High-Performance Computing (128)
- Hydropower (12)
- Irradiation (3)
- Isotopes (62)
- ITER (9)
- Machine Learning (67)
- Materials (156)
- Materials Science (156)
- Mathematics (12)
- Mercury (12)
- Microelectronics (4)
- Microscopy (56)
- Molten Salt (10)
- Nanotechnology (63)
- National Security (86)
- Neutron Science (169)
- Nuclear Energy (121)
- Partnerships (66)
- Physics (68)
- Polymers (35)
- Quantum Computing (52)
- Quantum Science (88)
- Security (30)
- Simulation (64)
- Space Exploration (26)
- Statistics (4)
- Summit (70)
- Transportation (102)
ORNL's Communications team works with news media seeking information about the laboratory. Media may use the resources listed below or send questions to news@ornl.gov.
1 - 10 of 12 Results
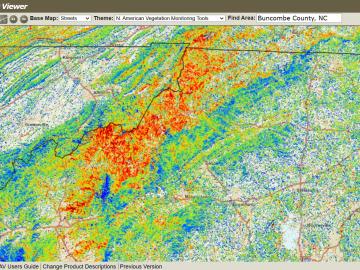
The ForWarn visualization tool was co-developed by ORNL with the U.S. Forest Service. The tool captures and analyzes satellite imagery to track impacts such as storms, wildfire and pests on forests across the nation.
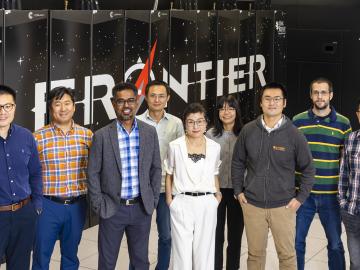
Researchers at Oak Ridge National Laboratory used the Frontier supercomputer to train the world’s largest AI model for weather prediction, paving the way for hyperlocal, ultra-accurate forecasts. This achievement earned them a finalist nomination for the prestigious Gordon Bell Prize for Climate Modeling.

ORNL researchers modeled how hurricane cloud cover would affect solar energy generation as a storm followed 10 possible trajectories over the Caribbean and Southern U.S.
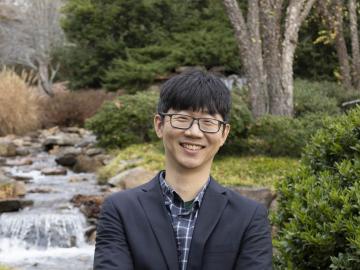
Lee's paper at the August conference in Bellevue, Washington, combined weather and power outage data for three states – Texas, Michigan and Hawaii – and used a machine learning model to predict how extreme weather such as thunderstorms, floods and tornadoes would affect local power grids and to estimate the risk for outages. The paper relied on data from the National Weather Service and the U.S. Department of Energy’s Environment for Analysis of Geo-Located Energy Information, or EAGLE-I, database.
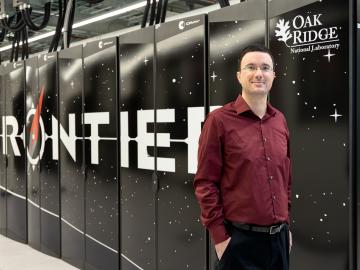
The Exascale Small Modular Reactor effort, or ExaSMR, is a software stack developed over seven years under the Department of Energy’s Exascale Computing Project to produce the highest-resolution simulations of nuclear reactor systems to date. Now, ExaSMR has been nominated for a 2023 Gordon Bell Prize by the Association for Computing Machinery and is one of six finalists for the annual award, which honors outstanding achievements in high-performance computing from a variety of scientific domains.
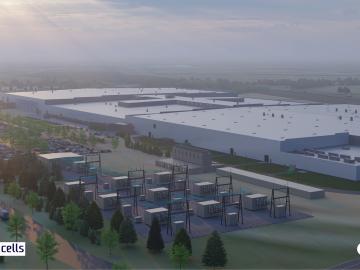
ORNL, TVA and TNECD were recognized by the Federal Laboratory Consortium for their impactful partnership that resulted in a record $2.3 billion investment by Ultium Cells, a General Motors and LG Energy Solution joint venture, to build a battery cell manufacturing plant in Spring Hill, Tennessee.

A novel method to 3D print components for nuclear reactors, developed by the Department of Energy’s Oak Ridge National Laboratory, has been licensed by Ultra Safe Nuclear Corporation.
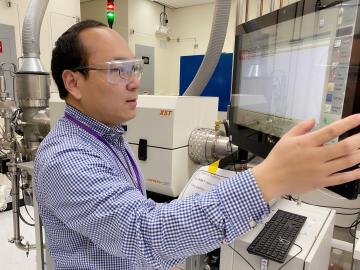
About 60 years ago, scientists discovered that a certain rare earth metal-hydrogen mixture, yttrium, could be the ideal moderator to go inside small, gas-cooled nuclear reactors.
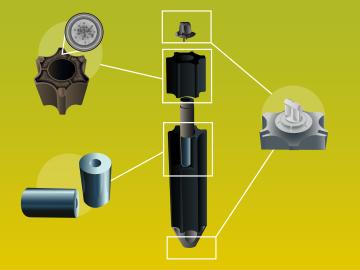
It’s a new type of nuclear reactor core. And the materials that will make it up are novel — products of Oak Ridge National Laboratory’s advanced materials and manufacturing technologies.

Oak Ridge National Laboratory researchers have developed artificial intelligence software for powder bed 3D printers that assesses the quality of parts in real time, without the need for expensive characterization equipment.