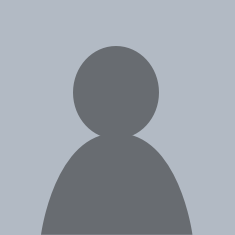
Bio
Sally Ghanem received the M.Sc. and PhD degree in electrical and computer engineering from North Carolina State University, Raleigh, NC, USA in 2021. Her research interests include the areas of computer vision, digital signal processing, image processing and machine learning.
Professional Experience
Oak Ridge National Laboratory
Machine Learning and Signal Processing Researcher
- Analyzed different color mappings by training on versions of a curated dataset collected in a controlled campus environment to investigate the effect of color space selection for visual inputs in vehicle recognition tasks.
- Constructed an efficient and robust wheel detector that precisely located and selected vehicular wheels from vehicle images. The associated hubcap geometry was utilized to extract fundamental signatures from vehicle images and exploit them for vehicle re-identification. Experiments showed that our approach could detect vehicular wheels accurately for 99.41% of the vehicles in the dataset.
- Developed a principled framework for decision fusion utilizing features extracted from vehicle images and their detected wheels. Siamese networks were exploited to extract key signatures from pairs of vehicle images. The proposed approach examined the extent of reliance between signatures generated from vehicle images to robustly integrate different similarity scores and provide a more informed decision for vehicle matching.
Awards
- Awarded Laboratory Directed Research and Development funding for the proposal titled “Vehicular Pattern of Life Analysis from Uncontrolled Multiple Views”, October 2023.
Received the best national laboratory collaboration award from National Nuclear Security Administration (NNSA), June 2019.
Education
North Carolina State University, Raleigh, NC
Doctor of Philosophy in Electrical and Computer Engineering
North Carolina State University, Raleigh, NC
Master of Science in Electrical and Computer Engineering
Professional Affiliations
Institute of Electrical and Electronics Engineers (IEEE)
Trademarks and Patents
- S Ghanem, RA Kerekes, RA Tokola, “Vehicle reidentification” - US Patent App. 18/139,172, 2023 (https://patents.google.com/patent/US20230343065A1/en)
- H Krim, S Roheda, S Ghanem, “Volterra neural network and method” - US Patent 11,842,526, 2023 (https://patents.google.com/patent/US11842526B2/en)
Publications
Other Publications
S. Ghanem, S. Roheda, and H. Krim. "Latent Code-Based Fusion: A Volterra Neural Network Approach." Intelligent Systems with Applications no. 18, Elsevier, 2023.
S. Ghanem, and H. Krim. "Scaling Subspace-Driven Approaches Using Information Fusion.", IntechOpen, 2023.
S. Ghanem, and R. A. Kerekes. "Robust Wheel Detection for Vehicle Re-Identification." Sensors 23, no. 1, 2022.
S. Ghanem, R. A. Kerekes, and R. Tokola. "Decision-Based Fusion for Vehicle Matching." Sensors 22, no. 7, 2022.
S. Ghanem, A. Panahi, H. Krim, and R. A. Kerekes. “Robust Group Subspace Recovery: A New Approach for Multi-Modality Data Fusion". IEEE Sensors Journal, 2020.
S. Ghanem, A. Panahi, H. Krim, R. A. Kerekes, and J. Mattingly. “Information subspace-based fusion for vehicle classification". In 26th European Signal Processing Conference (EUSIPCO), IEEE, 2018.
S. Ghanem, H. Krim, H. S. Clouse, and W. Sakla. “Metric Driven Classification: A Non-Parametric Approach Based on the Henze-Penrose Test Statistic". In IEEE Transactions on Image Processing, no. 12, IEEE, 2017.
S. Ghanem, E. Skau, H. Krim, H. S. Clouse, and W. Sakla. “Non-parametric bounds on the nearest neighbor classification accuracy based on the Henze-Penrose metric". International Conference on Image Processing (ICIP), IEEE, 2016.