ORNL researcher Ryan Kerekes leans on the original vehicle recognition system hardware, which required the large solar panels behind him, while holding the current version. His team has partnered with nonprofit RESOLVE to make the camera/sensor system lighter, more compact and more efficient. Credit: Carlos Jones/ORNL, U.S. Dept. of Energy
A technology developed to prevent poachers from killing endangered African species is being adapted by researchers at the Department of Energy’s Oak Ridge National Laboratory to recognize individual cars. The capability could help secure checkpoints, track nuclear materials and catch kidnappers.
“It’s like the face recognition on your phone, but for cars,” said Ryan Kerekes, who leads the project team in the lab’s Radio Frequency and Intelligent Systems group. “We needed a low-powered processor that was still capable of onboard, artificial-intelligence-based machine learning. It needed to be always vigilant, always processing, looking for a vehicle in each frame and determining if that vehicle could be cropped out and ‘fingerprinted’ for comparison with other images in its data bank.”
Researchers began the effort eight years ago by evaluating and testing the best types of sensors to recognize cars as they drive past, functioning in extreme environments with high security needs. The next step was developing the onboard software to compare footage of different cars, matching individual vehicles.
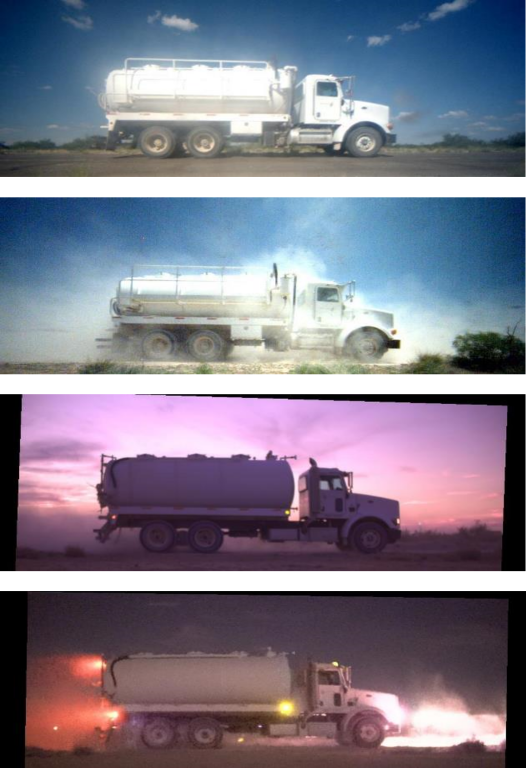
But when tested at checkpoints in Afghanistan, early versions of the technology proved complex and cumbersome, Kerekes said. The system included a desktop computer, cameras and sensors packaged in a 100-pound case, accompanied by two batteries the size of tissue boxes which often lasted less than a day. Charging them required six elevated solar panels mounted 15 feet in the air. The system worked, but it needed to be more portable and energy efficient.
The team combined forces with a nonprofit called RESOLVE that had developed a camera-alert system designed to photograph passing poachers in African national parks. “RESOLVE makes an intelligent trail camera with a motion sensor, all the size of a candy bar,” Kerekes said.
When triggered by a motion sensor, the RESOLVE TrailGuard AI system grabs the first images in 250 milliseconds and loads four frames into an internal microchip equipped with 14 microprocessors. The onboard artificial intelligence model recognizes human images and transmits them. The technology has also been used to prevent illegal rainforest logging, said Eric Dinerstein, director of biodiversity and wildlife solutions at RESOLVE.
RESOLVE is working with Kerekes’ team to adapt the technology for recognizing vehicles using an even faster chip that can support many detectors and a license plate-reading algorithm. Communications equipment housed in a case the size of a large wallet can send relevant images to a command center within 30 seconds, using mobile phone or satellite networks.
“Everything comes down to solving two problems: battery life and connectivity,” Dinerstein said. “One of the greatest contributions of the AI is saving on battery life because it doesn’t transmit false positives. We can get an average of 2,500 transmissions on a single charge that could last more than two years. That’s really a game changer for a remote sensor where you don’t want to be charging the battery every four months.”
Solving the hardware challenge was a vital step but useless without high-accuracy recognition software. ORNL researchers fine-tuned a neural network that chooses which vehicle features are most useful in making a particular match. These can include overall vehicle profile, hubcap shape, window size and placement. When comparing images, an algorithm rules out irrelevant differences, such as the way headlights illuminate the front of a vehicle at night. The system aims to recognize a truck down to its dents.
The technology could be used to track the location of specific vehicles, such as those known to be carrying nuclear materials. It can monitor whether a vehicle that enters a sensitive area emerges again at the time and place anticipated, Kerekes said. Police could use it to look for specific cars involved in kidnappings after an Amber Alert is issued. In the future, Kerekes is interested in adding radiation detection to the sensing platform, better allowing the system to monitor for unmarked vehicles carrying nuclear material across borders.
Kerekes said he is also exploring how the technology could be used to better prepare the U.S. power grid to integrate electric vehicles. By positioning camera sensors at the entrance to a neighborhood, utilities could identify how many electric vehicles are based there. Electric companies could use this information to understand where spikes might occur in the electricity load and to make decisions about where to upgrade equipment such as transformers. Kerekes’ team is testing this use of the technology by counting electric vehicles that pass the two gates to the ORNL campus.
The research was funded by the Defense Threat Reduction Agency, which enables the U.S. Government, the Department of Defense (DoD), and International Partners to counter and deter emerging threats and weapons of mass destruction.
UT-Battelle manages Oak Ridge National Laboratory for the U.S. Department of Energy’s Office of Science. The single largest supporter of basic research in the physical sciences in the United States, the Office of Science is working to address some of the most pressing challenges of our time. For more information, please visit energy.gov/science.