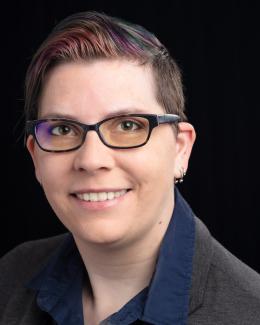
Bio
About Me
Dr. Andy Berres is a data scientist who specializes in data science at scale for urban applications. Their core expertise is data workflows, data fusion, analysis, geometry, and visualization, with additional experience in simulation, and machine learning. They work on on different platforms from web applications to high-performance computers. Dr. Berres has a passion for applied science, and has worked with a variety of applications ranging from plasma physics and medicine to climate science and transportation. As a research scientist in the Computational Urban Sciences group, they work primarily with large geographic and urban data. You can learn more about their current work in the projects section.
Before their time at ORNL, Dr. Berres was a postdoc in the Data Science at Scale group at Los Alamos National Laboratory where they worked on analyzing the connection between use of computational resources and cognitive value of the outcome by compressing image databases and evaluating the usability. They furthermore worked as a developer for MPAS-Ocean, the ocean component of the Energy Exascale Earth System Model (E3SM) project. During that time they extended multiple analysis members to enable the use of regional masks.
Dr. Berres completed their BSc (2009), MSc (2011), and PhD (2015) at the University of Kaiserslautern in Kaiserslautern, Germany. During their studies, they specialized in visualization, image processing, and computer vision. Their minors were in mathematics (BSc) and biology (MSc).
Current Projects
CTwin 2.0
Dr. Berres is leading the incident detection team and contribute to other data science and cyberphysical control efforts for the CTwin 2.0 Project. The project at large provides real-time and historic situational awareness and predictive capabilities for transportation in the Chattanooga area.
Occupancy Schedules
On this project Dr. Berres develops building occupancy schedules based on traffic data, building on previous work on coupled urban systems. This effort is part of the Population Density Tables project.
Urban-Scale Building Energy Modeling
As a member of the AutoBEM project, Dr. Berres is working on workflows for parallel simulation runs, for which they have run 125 million building simulations. A small extract of Clark County, Nevada is available on Zenodo, and a larger dataset comprising about 123 million buildings is available on Constellation.
SMART Mobility 2.0: Integrated Vehicle Speed Control
For this project, Dr. Berres is guiding the development of a cloud-based system for vehicle speed control, and in charge of developing vehicle-to-infrastructure communications. This system informs subscribed vehicles of signal timing changes and other data to support algorithmic and human-in-the-loop decisions about speed changes. This project is part of the Smart Mobility 2.0 Consortium, and it builds on the Real-Time Mobility Control System LDRD.
Previous Projects
Regional Mobility (CTwin)
Dr. Berres was responsible for leading the data science efforts for the Regional Mobility Project, which provided real-time and historic situational awareness for transportation in the Chattanooga area. They managed and analyzed data from over 100 different data sources. This data can come from sensors, probes, surveys, or other sources, with different spatiotemporal resolutions and availabilities. As part of this project, Dr. Berres led the development of various visualizations for the platform, which have been published (Visualization in Transportation presentation (video), Multiscale Visualization paper, Safety Visualization paper). This project is continued by the CTwin 2.0 project.
Real-Time Mobility Control System
Dr. Berres was also a member of an LDRD which uses similar data. For this LDRD, their main focus centered on analyzing and predicting signal timings, as well as supporting the design of a web-based platform to upload and view simulated traffic data. This project is continued by the SMART Mobility 2.0: Integrated Vehicle Speed Control project.
Coupled Urban Systems
Dr. Berres worked on the Coupled Urban Systems Project, which was a project under the Exascale Computing Project umbrella. During this project, they initialized a transportation simulation (TRANSIMS) using population and travel survey data, and coupled the outputs of this transportation simulation to a building energy simulation (EnergyPlus) by assigning occupants of vehicles to buildings that were near their destination. Furthermore, they have contributed to coupling the transportation output to an emissions model (MOVES). They contributed to two notable publications based on this project (Mobility-Driven Building Energy paper (led by Dr. Berres), Weather Impact on Traffic paper (led by Dr. Kuldeep Kurte)).
COVID-19
Dr. Berres contributed to the development of a web-based platform to present simulation outputs for various COVID-19 simulations, case count data, mobility data, and others. This work was funded by the Department of Energy under the CARES act. This work resulted in a paper about the platform itself (led by Dr. Gautam Thakur), with other publications in the pipeline.