Brief: Development of training data and artificial neural network potential for mechanics and fracture of materials.
Accomplishment: We propose a way to generate and select training data for their employment in simulations of deformation and fracture of crystals. We applied the proposed approach to monolayer 2D crystalline graphene, utilizing the density-functional tight-binding (DFTB) method for more efficient and extensive data generation in place of density functional theory (DFT). Based on this rich training data, we found that the data selection critically affects the accuracy of the developed artificial neural network potential (NNP), also indicating that relying only on total energies and atomic forces in the training data is insufficient to judge the NNP’s reliability. Therefore, we evaluate and select NNPs based on their performance in describing physical properties, e.g., stress-strain curves and geometric deformation. Our optimized NNP predicts straight crack propagation in graphene along both armchair and zigzag lattice directions, as well as higher fracture toughness of zigzag edge direction, showing agreement with previous experiments as opposed to bond order reactive potentials.
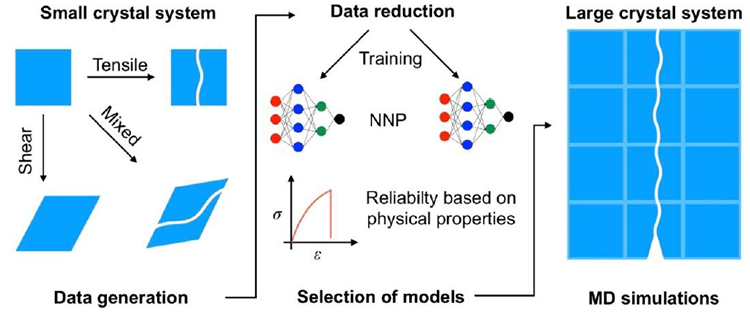
Acknowledgement: This research was partially supported by the AI Initiative, as part of the Laboratory Directed Research and Development Program of Oak Ridge National Laboratory, managed by UT-Battelle, LLC, for the U.S. Department of Energy (DOE). This research was funded by Wigner Fellowship at Oak Ridge National Laboratory and U.S. Department of Energy Fossil Energy and Carbon Management Program, Advanced Coal Processing Program, C4WARD project (FWP No. FEAA155).
Publications and presentations resulting from this work: Paper is under review at Nature Partner Journal Series (npj) Computational Materials.
Contact: Gang Seob Jung (jungg@ornl.gov)
Team: Gang Seob Jung, Myung Hoon Joo and Stephan Irle