Maxim Ziatdinov, a research scientist in the Computational Sciences and Engineering Division at the Department of Energy’s Oak Ridge National Laboratory, has been selected as a 2021 Foresight Fellow in Physics-Guided Artificial Intelligence for Molecular Machines.
Maxim Ziatdinov, a research scientist in the Computational Sciences and Engineering Division at the Department of Energy’s Oak Ridge National Laboratory, has been selected as a 2021 Foresight Fellow in Physics-Guided Artificial Intelligence for Molecular Machines. The honor was based largely on the potential of his ground-breaking work in “teaching” high-resolution microscopes, such as those at ORNL’s Center for Nanophase Materials Sciences, to recognize the atoms they observe and manipulate to them to assemble into useful structures.
The Foresight Fellowship is an exclusive one-year program offering support and mentorship to leading young scientists, engineers and innovators. The experience builds collaborative networks to accelerate bold ideas into transformative new technologies, with a focus on nanotech, biotech and computer science.
“The ultimate goal of nanotechnology was always to build things atom by atom from scratch, to design and build materials with the functionalities you want,” Ziatdinov said. There’s still work to be done in that regard, but he is bringing the goal steadily closer.
Sergei Kalinin is leader of the Data NanoAnalytics Group at CNMS. He said Ziatdinov was one of the first scientists worldwide to apply machine learning—a type of artificial intelligence—to electron and scanning probe microscopes, which can observe the behavior of atoms.
Electron microscopes rely on beams of electrons, rather than the beams of light in traditional microscopes, and have a resolution so high they can be used to observe atoms and record images that include hundreds of atoms at multiple frames per second. Scanning probe microscopes are sharp-tipped probes precise enough to sense the surface of objects at the atomic level and similarly generate tremendous volumes of data. But analyzing such a mass of data, a process that includes eliminating background “noise” from the images and identifying the locations and types of atoms, is difficult.
“In some sense, Maxim started the machine learning field in the area of electron and scanning probe imaging,” Kalinin said. “He has been delving in this area since 2015, barely four years after the first big successes of deep learning and well before present-day enthusiasm about these methods in the physics community.”
Ziatdinov had been working with both scanning probe microscopes and electron microscopy data, but the analysis procedure was so dependent on the skills, patience, and hunches of its human operator that the results were hard to reproduce.
His interest turned to automating the process by essentially replacing the human operator with an “intelligent” microscope programmed and trained to recognize different types of atomic structures in the images and tune out noise, allowing real-time analysis. Ziatdinov and his colleagues are honing this technique to make it more efficient, with the goal extracting as much information as possible about the atomic structures with the fewest possible measurements.
The next step is to teach the microscope to manipulate the atoms seen in the images, Ziatdinov said.
“Ideally you want to be able to harness this capability to assembling structures,” Kalinin said. “The vision we have, and that Maxim is at the forefront of implementing, is to teach the microscope to assemble the atoms in the way we want them to be assembled.”
This capability would represent a shift from observing matter on an atomic level to controlling matter on an atomic level. It’s part of Ziatdinov’s appeal to the Foresight Institute, said Kalinin, a past Foresight Fellow himself.
Ziatdinov’s expertise also demonstrates a powerful overlap between experimentation and machine learning. “Maxim laid the foundation for us,” Kalinin said. “He has become a bridge from microscopy, which is something ORNL is known for, to the quantitative physical sciences.”
Ziatdinov’s overlapping skill sets also impressed the Foresight Institute, a non-profit Silicon Valley think tank. While there are Foresight Fellows who design molecular machines and others who research artificial intelligence, Ziatdinov will be the first who combines hands-on research from both disciplines.
“I’m looking forward to collaborating with people from other disciplines who have been working for a while on trying to achieve similar goals in their respective fields,” Ziatdinov said. “I’ll be connecting with people who do fundamental research on autonomous systems, like self-driving cars.”
Such networking and mentorship can also help improve the pipeline between ORNL’s research and commercial applications.
Ziatdinov received his Ph.D. in materials science and engineering from Tokyo Institute of Technology in 2014, followed by a postdoctoral appointment at the Center for Nanophase Materials Sciences at Oak Ridge National Laboratory. He joined the research and development staff of the Computational Sciences and Engineering Division in 2018.
UT-Battelle manages ORNL for the Department of Energy’s Office of Science, the single largest supporter of basic research in the physical sciences in the United States. The Office of Science is working to address some of the most pressing challenges of our time. For more information, please visit energy.gov/science.
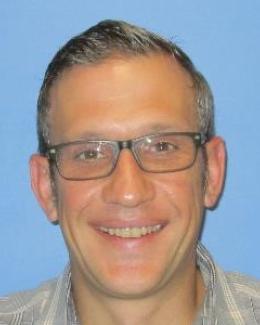