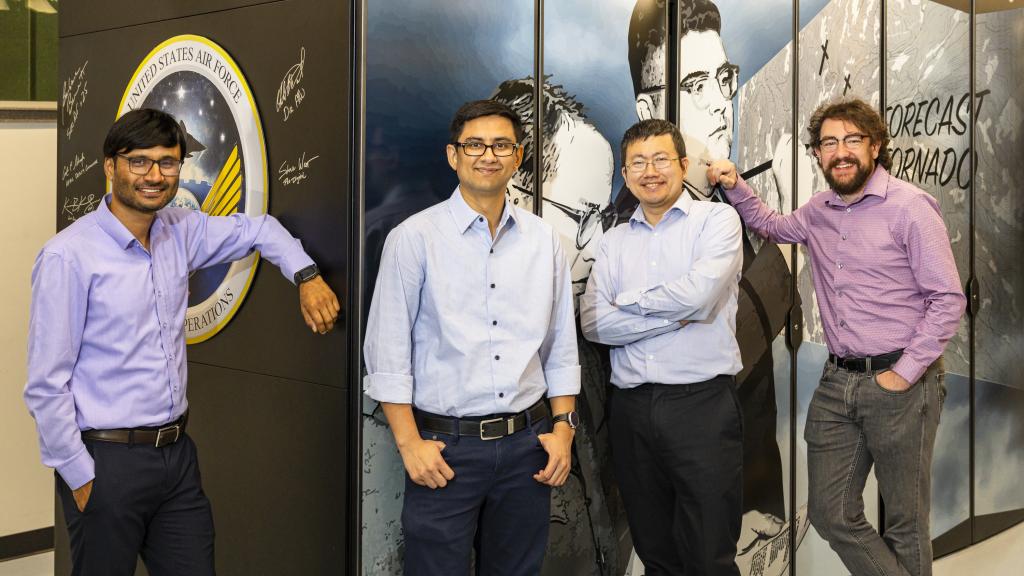
On Aug. 21, 2021, Waverly, Tennessee, received nearly one-third of its average annual rainfall in just a few hours — the resulting 17 inches of rain led to one of the deadliest flooding events on record.
In fact, Waverly faced some of the worst consequences of the severe rain and subsequent flooding that hit Central Tennessee in the summer of 2021. Unfortunately, the area was unprepared for the torrent that killed 21 people and caused over $100 million in property damage.
A combination of factors causes flooding, including rainfall, geographic location, the amount of moisture already in the environment, the flow of rivers and streams and surface runoff. And each factor has a direct impact on the severity of the flood. A variety of models can simulate these individually, but few studies have assessed the accuracy of a framework that captures the entire flood event.
To better understand the factors that contributed to the August 2021 floods, a multi-institutional team tested a new hierarchical flood modeling framework that can simulate the entire flood event and translate precipitation to inundation. One of the main questions with a multimodel approach is how errors propagate through coupled, or dependent, simulations.
In a study published in the Journal of Hydrology, researchers identified one of the most important sources of uncertainty, or error, in the flood models and demonstrated the accuracy and utility of the framework for predicting future floods.
The recent study explored a new approach for flood modeling that could improve predictions for future weather events.
The study was led by Sudershan Gangrade, Ganesh Ghimire and Shih-Chieh Kao from Oak Ridge National Laboratory’s Water Resource Science and Engineering group and Mario Morales Hernandez from the University of Zaragoza in Spain.
“We developed a modeling framework to unravel the August 2021 extreme flooding event at Waverly,” said Gangrade. “Developing and testing the framework for Waverly is not only useful for retrospective analysis but can also be utilized to simulate future flood scenarios.”
In this study, the research team used precipitation data to run two hydrologic models — the Variable Infiltration Capacity model and the Routing Application for Parallel Computation of Discharge, or RAPID — to simulate runoff and streamflow in the Waverly area. The outputs from those models were then fed into two inundation models — AutoRoute and the Two-dimensional Runoff Inundation Toolkit for Operational Needs, or TRITON, which is a GPU-optimized model designed to run on modern supercomputers.
The team wanted to know how inaccuracies in each model affected the final inundation models, which could be used to inform evacuation orders and emergency response.
To test the impact of different precipitation inputs on the final flood prediction, the team used both forecast data and observed data from the Waverly area. Running a model with data from a past weather event is a common practice known as hindcasting, which tests a model’s accuracy compared to observations.
“We wanted to perform a diagnostic evaluation of the Waverly flood,” said Ghimire. “We saw significant differences between the inundation model outputs from the forecast and observed precipitation data. The error in the precipitation forecasts propagated through the framework and was the main reason we underestimated the central Tennessee flood.”
Tests using the observed precipitation data showed that the framework accurately simulated the flooding, which led the team to conclude that errors in the inundation forecast primarily originated from the precipitation forecasts.
As forecasts improve and the team works to scale the models to cover larger domains within the U.S. and global watersheds, its usefulness in future flood preparedness and management holds real promise. The team is excited to explore opportunities to produce more accurate and timely predictions that can help communities better prepare for and respond to flood events.
This team also worked with researchers from the U.S. Army Engineer Research and Development Center, the University of Maryland, Tennessee Technological University and Follum Hydrologic Solutions, LLC. The work was supported by the U.S. Air Force Numerical Weather Modeling Program and ORNL’s Oak Ridge Leadership Computing Facility, a Department of Energy Office of Science user facility.
UT-Battelle manages ORNL for DOE’s Office of Science, the single largest supporter of basic research in the physical sciences in the United States. DOE’s Office of Science is working to address some of the most pressing challenges of our time. For more information, visit https://energy.gov/science. -- Betsy Sonewald
