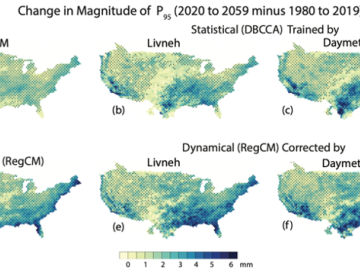
We present an intercomparison of a suite of high-resolution downscaled climate projections based on a six-member General Climate Models (GCM) ensemble from the 6th Phase of Coupled Models Intercomparison Project (CMIP6).
We present an intercomparison of a suite of high-resolution downscaled climate projections based on a six-member General Climate Models (GCM) ensemble from the 6th Phase of Coupled Models Intercomparison Project (CMIP6).
Multimodel ensembling improves predictions and considers model uncertainties. In this study, we present a Bayesian Neural Network (BNN) ensemble approach for large-scale precipitation predictions based on a set of climate models.
Important insights into many data science problems that are traditionally analyzed via statistical models can be obtained by re-formulating and evaluating within a large-scale optimization framework.
Spatial optimization seeks optimal allocation or arrangement of spatial units under constraints such as distance, adjacency, contiguity, and pattern. Evolutionary Algorithms (EAs) are well-known optimization heuristics.