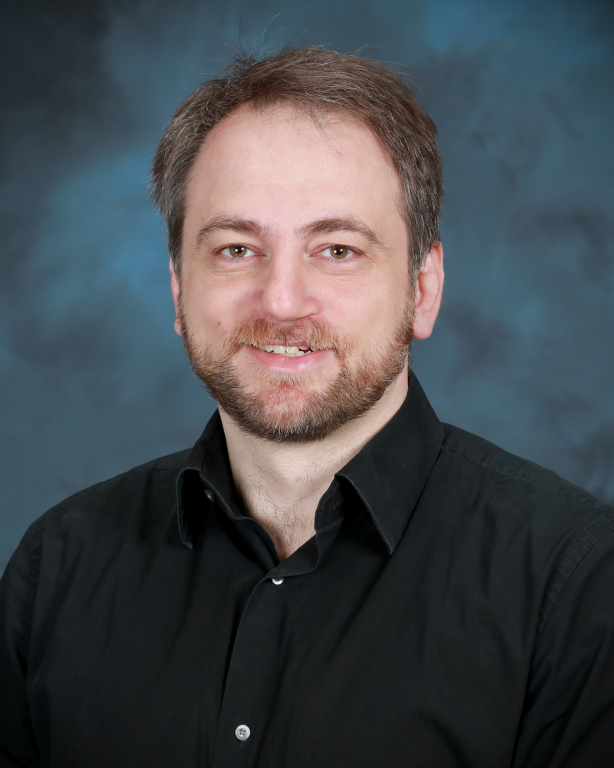
Artificial intelligence (AI) techniques have the potential to support medical decision-making, from diagnosing diseases to prescribing treatments. But to prioritize patient safety, researchers and practitioners must first ensure such methods are accurate.
In a 2019 perspective published in Nature Machine Intelligence, Edmon Begoli of Oak Ridge National Laboratory, Tanmoy Bhattacharya of Los Alamos National Laboratory, and Dimitri Kusnezov of the US Department of Energy discuss a need for and propose a path to establish safe, reliable AI practices for medical applications.
Specifically, they argue that applying the field of uncertainty quantification (UQ) to medical AI could revolutionize healthcare and save lives. UQ refers to the scientific process of predicting outcomes based on limited data to provide measures of confidence that are currently used to inform decisions vital to national and international security. Currently, no such systematic approach exists for AI.
With UQ, the authors anticipate that AI tools such as machine learning and deep learning could complete vital tasks such as analyzing and learning from datasets containing thousands of MRI scans with cancerous tumors to efficiently and accurately detect evidence of disease.
In the following interview, Begoli, Director of Scalable Protected Data Facilities in ORNL’s Computing and Computational Sciences Directorate, elaborates on this idea.
Q: What is UQ and what role could it play in the field of AI?
Begoli: UQ assesses the accuracy of computational models that influence important decision-making processes, aiming to quantify how much we can trust AI-generated decisions. This practice has had a particularly relevant application in the field of nuclear stewardship, in which supercomputer simulations of nuclear processes have replaced physical testing. UQ ensures that these models accurately reflect real-world events.
UQ can also inform emergency responses. For instance, weather simulations can predict the category of hurricanes, determine where they will hit, predict the amount of damage they will cause, and estimate how many people will be affected. UQ provides a degree of confidence needed to make critical decisions based on these simulations.
However, UQ has not yet been substantially applied to AI. In this article, we advocate for the necessity of developing UQ for medical AI. We know that UQ works in these other fields, and now we need to determine approaches and come up with principles for applying UQ to the AI field because physicians don’t currently trust the accuracy of models that can help diagnose patients. Modern AI can detect cancerous tissue from mammograms, brain scans, and other tests, as well as locate phenomena of interest in images and interpret clinical text, but certifying AI systems with UQ is a necessary step to avoid misdiagnoses and other potential errors.
Q: What areas of medicine stand to benefit the most from AI?
Begoli: There are dozens, if not hundreds, of medical areas where AI plays a significant role. It’s used for image-based diagnostics because deep learning methods excel at detecting anomalies in images. It’s also good at analyzing doctors’ notes and extracting relevant information from medical text and other clinical data by tracking patients over time to gain insights into cancer, Alzheimer’s, and other diseases. For example, we can use deep learning to track vital signs of hospital patients and detect when certain activity indicates the development of sepsis, a disease with a 50 percent mortality chance. These types of tools can help medical practitioners determine which patients to treat with antibiotics to prevent sepsis from developing.
Q: What is the current state of UQ in medical AI?
Begoli: It is in its infancy, but more and more research is emerging in the area, which could lead to advances in UQ for medical AI purposes. Ever since AI was first introduced, researchers have worked to develop decision support systems to help diagnose patients, but such tools have had a spotty adoption in practice because it’s difficult to guarantee that they won’t make mistakes. Just as physicians go through rigorous exams and years of training, UQ for AI would help prove the accuracy of AI systems that inform life-critical medical decisions.
Q: You write that UQ informs decisions related to risk management and nuclear stewardship. How far behind is medical AI?
Begoli: Very far behind. This state of affairs was one of our main motivations for writing this paper. It will not be possible to trust AI methods for broadly adopted clinical decision-making purposes without implementing UQ, which will require a concerted effort. We need to get physicians and mathematicians and computer scientists involved.
Q: What is the difference between a regular AI resource and one that incorporates UQ?
Begoli: AI is a black box because we don’t know exactly how algorithms are trained, but applying UQ would help in a couple ways. First, UQ would indicate how well the AI system is performing and how sensitive it is to certain datasets. Second, it might guide us to actually structure deep learning approaches to quantify errors, allowing an AI system to interpret its own architecture and track where things may go wrong. UQ would also provide a quality measure for AI that would be used as a metric for what is working and what is not, and we are now working on ways to implement that idea.
Q: How would enhanced UQ revolutionize healthcare?
Begoli: AI has tremendous potential, but there’s significant distrust surrounding the idea of applying AI to medicine. However, if trusted AI techniques can be implemented reliably on a global scale, it could help provide medical services to currently underserved populations.
For example, AI scanners can help diagnose vision loss resulting from diabetes and other diseases. These scanners take pictures to automatically detect the location and progression of degeneration in the eye by clearly showing where certain structures are starting to deteriorate through a process called macular degeneration. That helps save vision because these patients can then be prescribed injections or other effective, often inexpensive treatments.
And that’s just one example. Imagine having automated cancer detectors, where portable MRI scanners could diagnose cancer in individuals who live in remote areas without access to medical professionals such as radiologists and oncologists. Today, people live and die from cancer without even knowing they have it until it is too late.
AI automates processes and makes them more widely available, but it is not being used to its full potential in the medical field. We need UQ because having certified, trusted processes in place could lead to advances for important scenarios including early detection of diseases, better prenatal care, improved telemedicine, and reduction of medical errors and malpractice, to name a few.
Q: What is the most challenging aspect of applying UQ to medical AI?
Begoli: The absence of theory. Nuclear physics is a challenging field, but it is also one of the best understood areas because the mathematics behind it is well-understood. We have a mathematical formula that, at a theoretical level, can provide insights into how things look even before we see them for the first time. We have known what the general structure of the atom was before anyone had a chance to actually see it. Many fields have textbooks of theory that define how systems should behave, but that’s not true for AI. Instead, AI learns from data to make decisions, and there’s no exact theory that says how to make those decisions, which is a big challenge. It is difficult to build UQ on a foundation with so many unknown and data-sensitive components, so we have to pay very close attention to the limitations and dead ends.
Q: How can expertise at ORNL and DOE advance the development of UQ for medical AI?
Begoli: The first goal of the paper was to state why UQ is needed in AI, and the secondary goal was to implicitly state that the organizations such as DOE and its laboratories should play leading roles in this discipline because we have so much experience in UQ. DOE has expended significant time and effort to develop UQ for nuclear physics applications and has world-leading expertise in the field. ORNL also has substantial expertise in UQ as applied to uranium and nuclear reactor research, as well as its own focus on AI research through its AI initiative. Many large companies are working on AI, but not many of them are spending time on UQ, which provides an opportunity for DOE and ORNL to lead the way.
UT-Battelle LLC manages Oak Ridge National Laboratory for DOE’s Office of Science, the single largest supporter of basic research in the physical sciences in the United States. DOE’s Office of Science is working to address some of the most pressing challenges of our time. For more information, visit https://energy.gov/science.—Interview by Elizabeth Rosenthal
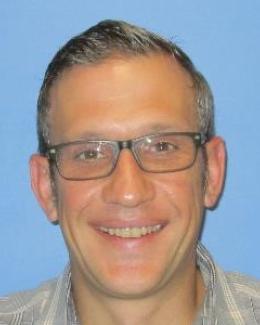