An ORNL team showed that process mining techniques could be used to make sense of immense health care databases such as the Department of Veterans Affairs’ Corporate Data Warehouse. Credit: Genevieve Martin/ORNL, U.S. Dept. of Energy
There are more than 17 million veterans in the United States, and approximately half rely on the Department of Veterans Affairs for their healthcare.
Successfully diagnosing, managing and treating such a massive number of patients is a tall order, yet a necessary one given the sacrifice made by the men and women of the American armed forces.
To improve its health data workflow and, by extension, patient care, the VA turned to the Department of Energy’s Oak Ridge National Laboratory, home to Summit, the nation’s most powerful supercomputer, and a wealth of expertise in computational and data science.
The VA’s health data system, known as the Corporate Data Warehouse, or CDW, is critical to monitoring patients’ progress. But it’s also immensely complex; imagine the massive amounts of data from 9 million patients as they seek and complete treatments for any number of issues that come and go over a lifetime.
To make sense of such a vast data resource, the ORNL team turned to process mining, which is the analysis of trends, patterns and details recorded by an information system. The team ultimately proved the technique could be used to make sense of immense databases such as the CDW.
While other health care studies have analyzed specific clinical departments or diseases, ORNL researchers were challenged to better understand how patients’ records moved through the entire system.
They focused on three specific tasks aimed at identifying cases where the treatment of the patient was not fully completed because of hidden issues in the information system. First, they had to decide which features to extract from the data, which was critical to the development of their analytical model; second, they had to format and normalize the data to accommodate the process mining approaches; and third, they had to perform the actual mining, which entailed applying several approaches to discover patterns in the data.
And because of the volume of data, the team was unable to complete an initial analysis of the full CDW database.
To make the data more manageable, the team focused on a cohort of more than 800,000 patients diagnosed with ischemic heart disease and extracted for study four different categories of care for analysis: consultation, radiology, laboratory services and prescriptions. For an idea of the scale of these data, the radiology dataset alone included more than 18 million events.
“We had to do a deep dive into features of the CDW and get familiar with the database quickly to decide what was relevant for our study,” said ORNL senior R&D staff member Hilda Klasky. “It’s a massive system and some data were definitely more important, for our purposes, than others.”
The team collected data values from all clinical orders of the four domains such as activities, statuses and dates, allowing them to apply process mining, develop process model maps and ultimately establish metrics such as frequency and performance for each domain.
“For instance, how many orders pass through the different steps, and how long does it take to go from one step to the next?” said Klasky. “We wondered if we could identify high-impact areas in the flow of clinical orders at VA.”
Their task was supported by the OASIS WS-Human Task Specification–State Transition Diagram, which defines all the steps necessary for a human task to be complete. By applying OASIS mapping to the data sequences they generated from the four categories, the ORNL team was able to tell what events in the raw sequence correspond to actual events, that is, determine which steps in a patient’s journey were completed and which ones failed.
“This was a data-driven study, and a massive effort due to the scale of the CDW,” said Ozgur Ozmen, ORNL R&D staff member. “The VA medical experts lent us their expertise, working with us to identify the critical data features, create the data models necessary to identify the anomalies, and further refine the approach.”
The team’s published work demonstrates that process mining, when combined with additional software tools, metrics and filters, can help with the examination of large volumes of data to address specific research questions for the CDW health care data. Beyond that, the collaboration also showed that powerful visualization tools can help to reveal trends in the data flow that might otherwise remain hidden and that the OASIS diagram can help rapidly identify unsuccessful case flows.
Moreover, the studies determined that about 80% of the radiology and consult orders follow the same path while the remaining 20% were observed to follow alternative, diverse paths. However, for laboratory and prescription orders, the inverse result was observed, demonstrating that most VA patients receive the full spectrum of care and providing a critical baseline from which to measure improvement.
Researchers are currently in the process of comparing the study’s results with the VA’s clinical departments’ workflows to check conformance of the process model maps generated from the data. This will assist them in identifying possible anomalies in the CDW database and improving care for the 9 million veterans who rely on the VA for their health care.
“Our collaboration with the VA was critical to the rapid evaluation of the more than 800,000 CDW records,” said ORNL senior R&D staff member Femi Omitaomu. “They were directly available to answer our questions and provide the domain knowledge needed to enable a rapid and efficient analysis capable of guiding the VA processes in the future.”
UT-Battelle manages ORNL for the Department of Energy’s Office of Science, the single largest supporter of basic research in the physical sciences in the United States. The Office of Science is working to address some of the most pressing challenges of our time. For more information, please visit energy.gov/science.
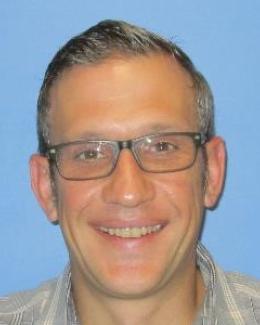